Data from wearable devices has been increasingly powerful in predicting various health conditions. New research from the University of Utah highlights how wearable sensors could be used to detect the key changes in our heart that allow us to predict heart failure days before a crisis occurs. It’s an ability that the researchers believe can help avert up to 30% of heart failure readmissions.
“This study shows that we can accurately predict the likelihood of hospitalization for heart failure deterioration well before doctors and patients know that something is wrong,” they say. “Being able to readily detect changes in the heart sufficiently early will allow physicians to initiate prompt interventions that could prevent rehospitalization and stave off worsening heart failure.”
The paper highlights that around 6.2 million Americans are currently living with heart failure, with the condition being the number one cause of hospital discharge diagnosis across the country. It’s estimated that around 30% of those patients will be readmitted within 90 days of discharge, and in many instances, the period in hospital actually diminishes the ability of the individual to care for themselves adequately.
“Those individuals who have repeated hospitalizations for heart failure have significantly higher mortality,” the researchers explain. “Even if patients survive, they have poor functional capacity, poor exercise tolerance and low quality of life after hospitalizations. This patch, this new diagnostic tool, could potentially help us prevent hospitalizations and decline in patient status.”
Heart failure
The researchers tracked 100 patients who had suffered from heart failure. They had an average age of 68, and were equipped with an adhesive sensor patch to be worn on their chest throughout the day for up to three months after their discharge. The sensor was designed to monitor their ECG and motion.
Data from the sensor was transmitted via Bluetooth to a smartphone app, from which it was then sent to an analytics platform for assessing the heart rate, respiratory rate, heart rhythm, activity and sleep levels, body posture and so on.
AI was then used to establish a baseline for each patient so that the system could detect when data deviated from the baseline, and could thus predict if the risk of heart failure was growing. It was able to do this accurately over 80% of the time, which resulted in predictions occurring over 10 days before readmission took place.
“There’s a high risk for readmission in the 90 days after initial discharge,” the researchers say. “If we can decrease this readmission rate through monitoring and early intervention, that’s a big advance. We’re hoping even in patients who might be readmitted that their stays are shorter, and the overall quality of their lives will be better with the help of this technology.”
The next stage is for the system to be tested via a large clinical trial alongside targeted, early interventions based upon the alerts to try and reduce reshospitalization rates.

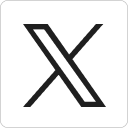



