The potential for AI in healthcare is something I’ve touched upon numerous times over the years, as indeed have I examined the numerous issues about the (ab)use of patient data in the pursuit of AI-driven improvements.
A new study from TUM and Imperial aims to better protect our personal medical data while still effectively training AI algorithms. The researchers describe how the technology has been put to the test in a system designed to spot pneumonia in x-ray images of children. It was able to produce similar results, in terms of accuracy, as existing algorithms that are not quite as hot on patient privacy.
Reliable data
There have been a large number of projects over the years that have showed the promise of AI in healthcare to provide faster and more accurate diagnoses. The quality of these systems depends on the quality of data used to train the algorithms. Traditionally, the more data researchers could get, the better the systems were, but this has obvious implications for patient privacy.
Most AI-based systems use anonymization to try and protect the identity of patients, but these have been shown to be easy to work around. The researchers believe that they have overcome this problem by producing an approach that maintains patient privacy while still giving researchers good data to work with.
“Guaranteeing the privacy and security of healthcare data is crucial for the development and deployment of large-scale machine learning models,” they say.
Protecting data
The researchers explain that one way of protecting medical records is to keep them physically on the same site they were collected on and not share them more widely. When clinics share patient data today, they often do so via copies of databases, which are sent to clinics to train their algorithms.
The researchers utilized an approach called federated learning, whereby a deep learning algorithm is shared rather than the actual data. The system was itself trained at a number of hospitals using local data before being returned to the authors. This means that the data owners weren’t required to share their data and maintained control.
“Our methods have been applied in other studies, but we are yet to see large-scale studies using real clinical data,” the researchers say. “Through the targeted development of technologies and the cooperation between specialists in informatics and radiology, we have successfully trained models that deliver precise results while meeting high standards of data protection and privacy.”
The researchers hope that their work, together with modern data protection methods, will support greater cooperation between institutions, especially given the privacy concerns that still riddle many AI projects today. For instance, earlier this year the NHS attracted the ire of privacy campaigners over the way in which they managed the opt-out process of co-opting medical records into a system to share them for research purposes.
“To train good AI algorithms, we need good data, and we can only obtain these data by properly protecting patient privacy,” the researchers conclude. “Our findings show that, with data protection, we can do much more for the advancement of knowledge than many people think.”

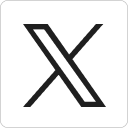



