A few years back I wrote about research into what it was like working under an “AI boss”. The paper found that while such an environment can be extremely productive, it can also be a dehumanizing affair. It was a situation replicated in a study by Alex Rosenblat into how Uber drivers feel about being managed by an algorithm.
A follow-up study from Penn State highlighted some clear areas for improvement for companies deploying algorithmic managers. It showed that while Uber’s AI performs many of the functions of a manager, drivers feel they have little ability to air grievances, pitch new ideas or even influence changes to their work, all of which would be possible with a human manager. This is compounded by the fact that most decisions made by Uber about their platform focus on the customer rather than the driver.
“All of Uber’s different management decisions are embodied in the platform as the company’s platform is actually doing the management,” the authors say. “When we looked at it, Uber’s platform seems to focus on one user — the person who wants a ride — somewhat at the expense of the drivers.”
Fair workplaces
This inherent sense of fairness is crucial, as it underpins how people feel when they’re managed by AI systems. A study from researchers at Carnegie Mellon found that by and large, people are quite happy to be managed by AI.
There is a but, however. People were happy with an AI boss so long as everything was running smoothly. If there were disagreements or things the employees wanted to change, then it resulted in a rapid deterioration in the relationship.
Research from Kellogg School of Management also shows how algorithmic management can influence the behavior of those under its charge. The study examined a gig economy platform that aims to help freelancers find work from around the world. As is common on these platforms, each freelancer is rated according to an algorithm that analyzes various factors relating to their performance in order to help buyers find the most suitable candidate.
Influencing behavior
The researcher went undercover on the platform and conducted interviews with both freelancers and the people hiring them. He also assessed formal and informal communication from the company and on their discussion forums.
It quickly became clear that freelancers were extremely anxious about their score and were concerned about it inexplicably going down. This fear was especially pronounced if the individuals had experienced such falls in the past, and especially so if they were dependent on the platform for income.
“Opaque third-party evaluations can create an ‘invisible cage’ for workers,” the researcher writes, “because they experience such evaluations as a form of control and yet cannot decipher or learn from the criteria for success.”
Lack of transparency
When the researcher first joined the platform the rating system was fairly transparent, in that freelancers were judged on the scores given for projects, with higher value projects given more weight in determining their overall score. When this approach failed to differentiate between freelancers, however, a more opaque algorithm was used instead.
The introduction of the algorithm significantly reduced the number of freelancers awarded the highest scores, and this helped to create a sense of paranoia around their scores, not least due to the lack of transparency around how the scores were constructed and how they might seek to improve them.
“What surprised me the most was that the highest performers and most experienced freelancers on the platform didn’t necessarily gain any advantage in terms of figuring out how the algorithm worked,” the researcher says. “Generally, those who do well in a system are able to figure out what’s going on to some extent. But in this context, even people whose scores hadn’t changed were very much on edge.”
Reacting to the system
Some freelancers reported that they tried to experiment in an attempt to understand how the system was rating them. Others, however, responded by constraining their activity in a bid to avoid the system entirely, such as by encouraging clients to move work off of the platform as quickly as possible.
The study suggests that the path taken by any given freelancer depended largely on the dependence on the platform for their income and whether they had experienced a fall in their score previously or not.
For instance, highly-rated freelancers who also got a lot of their income from the platform were heavily influenced by any recent fall in their score, with those freelancers tending to experiment to try and return their score to its former status. If no such fall had occurred, then freelancers would adopt the second strategy in order to try and protect their score.
The whole system is highly precarious, as while performance appraisals in traditional employment are designed, at least in part, to help elicit improvements in performance, on gig economy platforms they are designed largely to weed out the poorer performers and help buyers gravitate towards the top performers.
“For the platforms, it’s about them optimizing their overall dynamics; their primary goal is not to help workers improve,” the author says. “For people’s day-to-day lived experiences, especially when they’re relying on the platform for work, this can be very frustrating and difficult.”
As is so often the case, it is those who are most vulnerable and most dependent on the platforms that are most likely to be living in this “invisible cage”.
“The hope of bringing this invisible-cage metaphor to the forefront is to bring awareness to this phenomenon, and hopefully in a way that people can relate to,” the author concludes. “Of course, even when we become aware of it, it’s difficult to know what to do, given the complexity of these systems and the rate at which their algorithms change.”

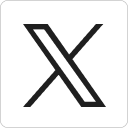



