The incredibly rapid pace with which the Covid-19 vaccines were developed was one of the most remarkable aspects of the pandemic, with numerous vaccines developed in a fraction of the time normally required.
Researchers from MIT’s Computer Science and Artificial Intelligence Laboratory (CSAIL) explored how machine learning can further support these efforts and help us find effective drugs even faster. The researchers highlight that Covid presents a challenge as it requires the virus to slow down and the data we have on it to speed up in order to effectively develop treatments that keep pace with any mutations.
Using data
Ordinarily, when data is used to help develop new drug combinations, it requires huge quantities in order to be effective. This has obvious limitations when data itself is limited. As such, the team developed a neural network that aims to learn both the drug-drug synergy and the drug-target interaction to come up with possible new combinations.
The model then tracks the interaction between a drug and known biological targets that the researchers identified as being related to the disease. The model then learns the antiviral activities of the drug via determining the virus yield in any infected tissue cultures. Through this, the team believes the model can predict the synergy of any two drugs.
The approach yielded a couple of new drug combinations, including remdesivir and reserpine, and remdesivir and IQ-1S, both of which seemed to produce good results against the virus.
“By modeling interactions between drugs and biological targets, we can significantly decrease the dependence on combination synergy data,” the researchers say. “In contrast to previous approaches using drug-target interaction as fixed descriptors, our method learns to predict drug-target interaction from molecular structures. This is advantageous since a large proportion of compounds have incomplete drug-target interaction information.”
The hope is that by using a combination of drugs it will be possible to maximize the efficacy of the treatment while also ensuring minimal side effects. What’s more, by using such a mixture, it also has a greater chance of minimizing the risk of resistance to the treatment, as many of these resistant mutations are mutually exclusive.
If the model proves successful with Covid, the researchers hope that it can be similarly effective both on other variants of the virus and also other diseases altogether. They plan to continue developing the model and hope to incorporate extra information, including the interaction between proteins and the way genes regulate themselves.

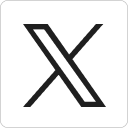



