Mammograms are undoubtedly crucial in the fight against breast cancer, but critics nonetheless argue that they’re expensive and prone to providing false-positive results. Advocates argue that personalized screening would help to overcome this challenge, and researchers from MIT’s Computer Science and Artificial Intelligence Laboratory (CSAIL) believe that their AI-based solution could take us further in that direction.
This resulted in the development of Tempo, which uses an AI-based risk model to help produce better screening guidelines. The model was training using a huge dataset of mammograms from Massachusetts General Hospital (MGH), before being tested on patients from the same hospital. The results suggest similar efficacy in terms of early detection but while requiring 25% fewer tests than is otherwise the case.
“By tailoring the screening to the patient’s individual risk, we can improve patient outcomes, reduce over treatment and eliminate health disparities,” the researchers say. “Given the massive scale of breast cancer screening, with tens of millions of women getting mammograms every year, improvements to our guidelines are immensely important.”
Personalized screening
Currently, screening is done according to age, with the population split into a few relatively large groups, such as those over 55, with recommendations the same for everyone in each group. The researchers believe that their model allows for much more personalized recommendations to be made.
“A key aspect of these models is that their predictions can evolve over time as a patient’s raw data changes, suggesting that screening policies need to be attuned to changes in risk and be optimized over long periods of patient data,” they explain.
The Tempo model aims to dynamically adapt so that changes to the screening frequency for each patient can be updated according to the changing risk profile of the individual. The system uses a simple metric for early detection and assumes that the breast cancer can be spotted around 18 months in advance.
While the team accepts that their work is by no means perfect, they hope that further developments in their tumor growth models could help it to successfully operate with a range of different tumor characteristics.
“Our framework is flexible and can be readily utilized for other diseases, other forms of risk models, and other definitions of early detection benefit or screening cost. We expect the utility of Tempo to continue to improve as risk models and outcome metrics are further refined. We’re excited to work with hospital partners to prospectively study this technology and help us further improve personalized cancer screening,” the researchers conclude.

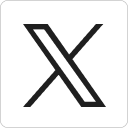



