The online rating system is crucial to the gig economy, with workers typically thriving or failing based upon their rating. Of course, such ratings are often a pretty blunt instrument when it comes to truly assess someone’s skills and capabilities.
What’s more, given the financial implications of the ratings, it’s not even possible for workers to shrug off poor ratings as insignificant, with nearly all platforms using workers’ ratings to determine not only their visibility on the platform but also their eligibility for incentives and even their continued employment.
Outsourced management
Such is the power of the rating system that the platforms have effectively outsourced the management of workers to the algorithms behind the ratings. The rise of these “digital bosses” is chronicled in a recent paper from the Kellogg School of Management. The authors attempt to explore how workers react and attempt to resist the power of the ratings, whether through pre-vetting customers or even dropping work in the middle of a project to fend off a potentially bad rating.
The authors divided the interaction between the worker and the customer into three stages, which define the period before the task, during the task, and then after the task. Each of these stages generated quite distinct actions from the worker to try and reclaim a degree of control over proceedings, and especially over how they’re rated. As the work progresses through each stage, however, the ability of the worker to resist unfair ratings dwindled, with any attempts made to do so generally burning out the worker involved.
While this has obvious implications for the workers involved, the researchers believe that it also serves up problems for the platforms too. This is because the interests of the customer are not always aligned with the interests of the platform, especially as customers are rarely held to account for the ratings they provide.
Gig economy
The researchers assessed the interplay between workers and clients via platforms they refer to as “FindWork” (an UpWork style platform) and “RideHail” (a ride sharing platform). The researchers both spent a number of years actually working on each platform, while also conducting interviews with workers and customers.
The analysis revealed some clear patterns. For instance, as workers began to move through the various stages of a job, they would typically respond with specific resistance measures during each stage, with their power to push back diminishing as they moved through the stages.
For instance, during the initial stage, workers would often perform considerable research into potential customers and try to vet them through both their profiles and the initial conversations with them. If a customer has a track record of giving low ratings, for example, this would often prove a sufficient red flag to put off working with them. Some workers would even demand a 5-star rating from a customer before even agreeing to work with them.
On the job
When workers were completing the task this understandably diminished their ability to resist any demands placed on them by the customer. At this stage, it was inevitable that the worker would be rated by the customer, and both parties were aware of this fact.
The researchers reveal that by this stage, workers have to deploy different tactics, such as potentially offering a discount in return for a high rating. Some workers would also request that a job be divided into smaller contracts so that they could gain multiple ratings for a single project, with this tactic especially common for clients they trusted.
If things were not going well, workers would commonly cancel the project early, as while this meant they wouldn’t get paid, it also meant they wouldn’t have to run the risk of a poor rating. The authors explain that with no manager or other mediating force to liaise with, workers have to fend for themselves as management has essentially been outsourced to the algorithm. This results in various strategies to try and mitigate any risks involved.
Hail Mary
This leaves the final stage, which is where workers have the fewest options to push back against any ratings they receive. This lack of options leaves many resorting to quite desperate measures, such as officially filing a dispute with the platform or retaliating by giving the customer themselves a similarly low rating.
These tactics are largely futile in terms of getting their own low rating changed but are often the only methods available to the worker so they at least give a degree of agency to them. The authors believe that the frustrations with the rating system are beginning to produce problems for the platforms as well as workers.
They argue that there is growing discontent about the treatment of workers by the platforms, together with a growing willingness to band together to try and strike a better bargain and pushback against the fundamental business model of the platforms.
With the kind of rating systems used by the platforms becoming more pervasive in a wider range of settings, however, the researchers believe this may be a problem that will extend far outside the boundaries of traditional gig workers in the years ahead. They argue that it is perhaps time to rethink just what the outsourcing of managerial control of workers to customers does to the relationship between employer and employee and ask ourselves whether it’s really a road we want to go down.

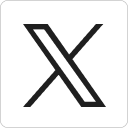



