Many of the advances we’ve seen in AI in recent years has been due to the availability of enormous open-source databases to help train the algorithms. Research from UC Berkeley warns, however, that when these datasets are used “off label” and applied in unintended ways, a range of biases can be introduced that compromise the very integrity of the system.
The results highlight the risks inherent when data intended for one task is used to train algorithms for a different one. The researchers discovered the issue when they struggled to replicate promising early results from a medical imaging study.
Help and hindrance
Free online databases have proliferated over the years, and they’ve considerably helped with the development of AI algorithms, especially in areas such as medical imaging. For instance, the ImageNet database has millions of images, which are used to train AI systems to successfully decode the measures contained within a scan. What many AI researchers may not understand, however, is that the files in these databases may be preprocessed rather than raw.
Raw image files typically contain far more data than their compressed counterparts, so it’s important that AI is trained on raw files as much as possible. The availability of raw databases is rare, however, so developers often make do with processed scan images.
“It’s an easy mistake to make because data processing pipelines are applied by the data curators before the data is stored online, and these pipelines are not always described. So, it’s not always clear which images are processed, and which are raw,” the researchers explain. “That leads to a problematic mix-and-match approach when developing AI algorithms.”
Performance bias
The study explains how this can result in performance bias by applying three well-known algorithms that reconstruct MRI images. They used the algorithm on both raw and processed images taken from the fastMRI dataset. The algorithm was nearly 50% better when using processed data than from images produced from raw data.
The researchers questioned whether these results were too good to be true, however, so took raw files and used two common data-processing pipelines that are known to affect open-access MRI databases: data storage with JPEG compression and the use of commercial scanner software. Three image reconstruction algorithms were trained using these datasets before their accuracy was measured.
“Our results showed that all the algorithms behave similarly: When implemented to processed data, they generate images that seem to look good, but they appear different from the original, non-processed images,” the researchers say. “The difference is highly correlated with the extent of data processing.”
Excessive optimism
The researchers then explored the risk that using these pre-trained algorithms in a clinical environment might pose. They took the algorithms that had already been trained on the processed data and then applied them to raw data from the real world.
“The results were striking,” the researchers explain. “The algorithms that had been adapted to processed data did poorly when they had to handle raw data.”
In other words, while the images may look fine, they are nonetheless inaccurate, with sometimes crucial clinical details missing. So while the algorithms seem to do well in test environments they fail to reproduce these results with raw data.
“No one can predict how these methods will work in clinical practice, and this creates a barrier to clinical adoption,” the authors conclude. “It also makes it difficult to compare various competing methods, because some might be reporting performance on clinical data, while others might be reporting performance on processed data.”

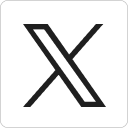



