Electronic medical records are both a vital part of modern healthcare systems and also the bain of many clinician’s life. In the US, the government has mandated that records are digitized, with the aim being to improve patient care.
Things are seldom so straightforward, however, as the data in the medical records is often extremely messy and contains a lot of jargon and abbreviations. A big part of the problem is that the notes are ostensibly written with humans in mind rather than machines, so computers have difficulty extracting any useful information from them to train any AI-based systems. There are also challenges in getting AI systems that work in one hospital to work well in another.
A way forward
Researchers from MIT’s Computer Science and Artificial Intelligence Laboratory (CSAIL) believe that they’ve found a way to do that. Their approach relies on large language models to both work in multiple hospitals and learns from various types of information. The model they developed used data from a huge GPT-3 style model and was able to expand on jargon, acronyms, and medication regimens.
Medical data comes with many challenges associated with it, not least of which are the various regulations that surround using it for training AI systems. This resulted in the researchers developing their own data via publicly available clinical datasets.
“It’s challenging to develop a single general-purpose clinical natural language processing system that will solve everyone’s needs and be robust to the huge variation seen across health datasets,” the researchers explain. “As a result, until today, most clinical notes are not used in downstream analyses or for live decision support in electronic health records. These large language model approaches will completely transform clinical natural language processing.”
The team plans to continue developing their model so that it incorporates other languages, while also working to quantify any uncertainty in the model. They also want to ensure that similar results can be achieved with the various open-source models on the market.
“Clinical information buried in unstructured clinical notes has unique challenges compared to general domain text mostly due to large use of acronyms, and inconsistent textual patterns used across different healthcare facilities,” they conclude. “To this end, this work sets forth an interesting paradigm of leveraging the power of general domain large language models for several important zero-/few-shot clinical NLP tasks. Specifically, the proposed guided prompt design of LLMs to generate more structured outputs could lead to further developing smaller deployable models by iteratively utilizing the model generated pseudo-labels.”

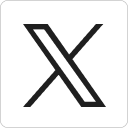



