Few AI-based tools have made such a splash as that made by chatGPT in recent months, with commentators breathlessly proclaiming that all manner of professions will be upended by the tool, its peers, or the technology that underpins them. There’s a perception that the introduction of AI technology into the workplace is a good thing, but in an article last year, I examined whether this is really the case.
The article was based on research questioning whether we tend to value things in humans that AI is also very good at, such as conscientiousness. Research from the University of Manchester casts additional doubt on the merits of AI in the workplace.
Making work smarter
The researchers question the assumption that AI will streamline our work to such an extent that many jobs will be rendered obsolete entirely. When analyzing the introduction of AI into a number of laboratories, however, the researchers found that simplification was not the default outcome, with some tasks made far more complex as a result, with a wide range of new tasks created.
The scientists were all engaged in work around synthetic biology, which aims to redesign organisms so that they have new abilities. It usually involves things like growing meat in a lab, discovering new drugs, and finding innovative ways of producing fertilizers.
Experiments in the field usually rely heavily on robotic platforms to move large numbers of samples autonomously and repetitively. They also heavily use machine learning in the analysis of large-scale experiments. This analysis then also produces large quantities of digital data as the various tools available have transformed how research is undertaken.
Time saving
The promise of such automation is clear in that it should enable scientists to both massively scale up their work while also saving them time that they can then devote to other tasks. The reality, however, was not quite so clear-cut.
The researchers found that the scientists were not really freed from the boring, mundane, and repetitive tasks in the way that they would have hoped. Instead, those tasks were both amplified and diversified as a result of the new technology.
For instance, there was a significant increase in the number of experiments and hypotheses that had to be performed, with automation amplifying this increase. While on the one hand, this is good, as it allows more hypotheses to be tested and also a greater number of tweaks to the experiments could be made, it also greatly increased the amount of data to be checked, standardized, and shared.
Training the helpers
What’s more, the robots also needed to be adequately trained so that they could effectively perform the tasks required of them. The scientists also needed to be trained to work effectively so that they could function alongside the robot. This involved learning how to prepare, repair, and supervise the machines.
Evaluation of scientific work is frequently based on outcomes such as grants and peer-reviewed publications. Nevertheless, the time and effort invested in cleaning, troubleshooting, and supervising automated systems often conflict with the activities traditionally recognized in the scientific community. These tasks, which are considered less valuable, may also go unnoticed because managers are less involved in the laboratory work and are not aware of the routine tasks.
Synthetic biology scientists who perform these duties are not compensated better or granted more autonomy than their managers. Moreover, they perceive their workload as heavier than those higher up in the job hierarchy.
Careful implementation
The research reminds us that introducing AI and other automation technologies may not produce the labor savings that we expect. Indeed, Tomas Chamorro-Premuzic argues in his latest book that often we become slaves to the algorithm rather than the other way around as our lives become devoted to generating the kind of data the algorithms need to perform.
Similarly, various language tools, such as ChatGPT, were developed with the help of a huge army of “ghost workers” who were paid peanuts to fine-tune and develop these tools for public use.
This exemplifies the invisible work required for the development and maintenance of digital infrastructure, which is known as the “digitalization paradox.”
The assumption that automation and digitalization lead to increased productivity and free time for all involved or affected is challenged by this phenomenon. Organizational and political efforts to automate and digitalize work are motivated by concerns about productivity declines. However, we must not unquestioningly accept claims of increased productivity gains.
Instead, we must examine our productivity metrics and acknowledge the unseen tasks that people perform, in addition to the more visible work that is typically rewarded. Moreover, we need to ensure that technology supports human capabilities by designing and managing these processes effectively.

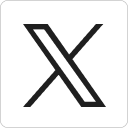



