Despite being hailed as impartial, objective evaluations of risk, crime, and likelihood of reoffending, computer-based algorithms were intended to eliminate the disparities and prejudices inherent in human decision-making across a range of applications, including law enforcement, bail, sentencing, and parole. However, up to this point, these algorithms have not lived up to their promise.
The Bureau of Justice Statistics, which operates under the umbrella of the US Department of Justice, reported that in 2021 (the most recent year for which data is available), there were 1,186 Black adults and 1,004 American Indians and Alaska Natives incarcerated in state or federal facilities for every 100,000 adults. In contrast, the rate of incarceration for white individuals in the same year was significantly lower at 222 per 100,000.
Algorithmic help
Recent research from Boston University explores the role algorithms play in delivering these unfair outcomes. While we previously thought of algorithms as impartial and unbiased, we’re increasingly aware that they often have our own biases hard-coded into them.
Imagine a scenario where a judge is provided with a recidivism risk score that has been generated through an algorithm as part of a report on a convicted criminal. This score serves as an indication of the probability that the individual will commit another offense in the near future. The judge uses this score as a factor in their decision-making process and hands down a longer sentence to someone with a high recidivism score. The case is then considered closed.
The author identifies three causes for this problem. Firstly, jurisdictions often fail to be transparent about their implementation and utilization, and frequently introduce them without seeking input from marginalized communities, who are most impacted by their use. Secondly, these communities are usually excluded from contributing to the development of these algorithms. Lastly, even in jurisdictions where public feedback is accepted, it is uncommon for it to result in any meaningful changes to the implementation of such tools.
Marginalized groups
This can result in racially marginalized groups being excluded from the very outset of the development of these algorithms.
“I’ve been looking at the decision-making power of whether and how to use algorithms, and what data they are used to produce. It is very exclusionary of the marginalized communities that are most likely to be affected by it, because those communities are not centered, and often they’re not even at the table when these decisions are being made,” the author explains. “That’s one way I suggest that the turn to algorithms is inconsistent with a racial justice project, because of the way in which they maintain the marginalization of these same communities.”
Not only do algorithms tend to generate biased outcomes that disproportionately affect underprivileged communities, but the data used to train these algorithms can also be disorderly, subjective, and prejudiced. Indeed, while we often think that the data used to train the algorithms is purely quantitative, the reality is usually very different.
Policymakers collaborate with computer engineers and data designers to identify the specific issue that their algorithm should address, as well as which datasets to utilize in its development. For instance, in the context of law enforcement and justice, this could entail collaborating with judges to establish what information would enable them to make more informed decisions about sentencing.
However, it is less probable that data engineers would seek input from incarcerated individuals as part of their initial information-gathering process.
Garbage in
The vast majority of extensive datasets employed in pretrial algorithms are constructed from information obtained from “carceral knowledge sources,” such as court records and police reports.
According to the author, genuinely fulfilling the potential of algorithms in the criminal justice system, which is to create a more standardized and impartial process than what humans are capable of, necessitates a comprehensive overhaul of the existing system. She urges her students to reflect on this as they work to shape the future of the legal and criminal justice fields.
“It means actually accounting for the knowledge from marginalized and politically oppressed communities, and having it inform how the algorithm is constructed,” she concludes. “It also means ongoing oversight of algorithmic technologies by these communities, as well. What I am contending requires building new institutional structures, it requires shifting our mindset about who is credible and who should be in power when it comes to the use of these algorithms. And, if that is too much, then we can’t, in the same breath, call this a racial justice project.”

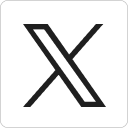



