Research conducted by ESMT Berlin reveals that while machines are capable of making superior decisions compared to humans, humans often struggle to recognize when a machine’s decision-making is more accurate. This tendency to override algorithmic decisions to the detriment of outcomes is referred to as algorithm aversion.
While algorithm aversion has been commonly attributed to a fundamental mistrust of machines, this study introduces a new perspective. The research highlights that the context in which human decision-makers operate can also hinder their ability to discern whether machines generate better recommendations.
Working together
To investigate the circumstances under which a human decision-maker, responsible for supervising critical machine decisions, can effectively assess the quality of machine-generated recommendations, the researchers developed an analytical model.
In this model, a human decision-maker oversaw a machine entrusted with vital choices, such as determining whether a patient should undergo a biopsy. Subsequently, the human decision-maker made the optimal selection based on the information provided by the machine for each task.
The study found that when a human decision-maker followed the machine’s recommendation and achieved a positive outcome, their trust in the machine increased.
However, in situations where the human decision-maker did not observe the correctness of the machine’s recommendation, such as when they chose not to pursue further actions, trust remained unchanged, and no valuable lessons were gained.
Assessing the machine
This interplay between the human’s decision and their assessment of the machine engenders biased learning. Consequently, over time, humans may fail to acquire the proficiency needed to effectively utilize machines.
These findings clearly demonstrate that the tendency to override algorithmic decisions is not solely driven by an inherent mistrust of machines. Rather, the accumulation of biased learning, facilitated by consistent overriding, can lead to the erroneous and inefficient deployment of machines in decision-making processes.
“Often, we see a tendency for humans to override algorithms, which can be typically attributed to an intrinsic mistrust of machine-based predictions,” the researchers explain. “This bias, however, may not be the sole reason for inappropriately and systematically overriding an algorithm. It may also be the case that we are simply not learning how to effectively use machines correctly, when our learning is based solely on the correctness of the machine’s predictions.”
The findings remind us that people often lack sufficient trust in technology’s ability to make effective decisions, but without this, we can’t really effectively utilize the capabilities technology has.
“Our research shows that there is clearly a lack of opportunities for human decision makers to learn from a machine’s intelligence unless they account for its advice continually,” the authors conclude. “We need to adopt ways of complete learning with the machines constantly, not just selectively.”

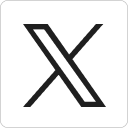



