Artificial intelligence can be a valuable tool for enhancing productivity and reducing costs, but it carries a troubling drawback: It often reflects the biased and discriminatory content found in the vast data it learns from the internet. New research from the Haas School of Business emphasizes the need for creators of AI models to be acutely vigilant in addressing these biases.
The researchers stress that people, with their inherent biases, significantly influence the development of these models. Understanding how human behavior and psychology affect the creation of these valuable tools is crucial.
How biases emerge
In their recent paper, the researchers draw on lessons from social psychology to explore how bias emerges and what can be done to combat it.
The bias issue begins with the data used to train AI systems. This data frequently contains stereotypes and can either marginalize or completely overlook certain groups, resulting in “representation bias” that defaults to a white, male, heterosexual perspective.
The problem is compounded by the fact that AI engineers often use annotators—humans who review and categorize data. Without a deliberate focus on achieving fair representation, certain groups may be unintentionally excluded, leading to biased outcomes in the AI model.
Furthermore, programmers themselves are not immune to their own implicit biases. Those constructing AI models are often in privileged positions, which can enhance their sense of psychological power and reinforce biases.
Now is the time
The researchers suggest a critical juncture in addressing this issue. One path is to continue using these models without addressing their flaws and relying on computer scientists to mitigate bias on their own. Alternatively, they propose collaboration between experts in biases and programmers to combat racism, sexism, and other biases in AI models.
To make progress, programmers and their managers should undergo training to become aware of their biases and take steps to account for data gaps or stereotypes when designing models. Additionally, the field of AI fairness has emerged, employing complex mathematical formulas to ensure that machine learning systems treat different groups equally based on factors like gender, ethnicity, sexual orientation, and disability.
Organizations can support their models by educating programmers about algorithmic fairness tools, such as IBM’s AI Fairness 360 Toolkit, Google’s What-If Tool, Microsoft’s Fairlearn.py, or Aequitas. Since each model is unique, organizations should collaborate with experts in algorithmic fairness to understand how bias might manifest in their specific programs.
In a broader context, companies can foster a culture of bias awareness in AI, allowing individual employees to report biased outcomes and supporting them in addressing these issues with their supervisors. This collaborative effort is essential as AI continues to become more prevalent, and until programming advances to create better models, organizations play a pivotal role in improving the fairness of AI model outcomes.

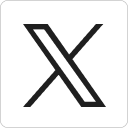



