The lack of diversity in the data used to train AI-based systems has long been a cause of concern. A study from the University of Michigan checking how fair OpenAI’s CLIP is found it doesn’t do well with images showing low-income and non-Western lifestyles. CLIP is the tech behind the DALL-E image generator.
“During a time when AI tools are being deployed across the world, having everyone represented in these tools is critical. Yet, we see that a large fraction of the population is not reflected by these applications—not surprisingly, those from the lowest social incomes. This can quickly lead to even larger inequality gaps,” the researchers explain.
Homogenous data
Models such as CLIP are like building blocks for AI, trained on lots of unlabeled data to work for various things. If these models learn from biased data, that bias can spread to other tools and applications that depend on the AI.
“If a software was using CLIP to screen images, it could exclude images from a lower-income or minority group instead of truly mislabeled images. It could sweep away all the diversity that a database curator worked hard to include,” the researchers continue.
The team tested how well CLIP performs using Dollar Street, a diverse image collection from Gapminder Foundation. This dataset has 38,000+ images from homes with different incomes across Africa, the Americas, Asia, and Europe. Monthly incomes in the dataset go from $26 to almost $20,000. The images show everyday stuff, annotated with topics like “kitchen” or “bed.”
Rating images
CLIP rates how well text and images go together, creating a score. This score gets used in other apps, like flagging or labeling images. DALL-E, an OpenAI model, relies a lot on CLIP. The researchers checked CLIP’s bias by scoring how well it matched images and annotated text, then seeing if the CLIP score related to household income.
“We found that most of the images from higher income households always had higher CLIP scores compared to images from lower income households,” the researchers explain.
Take the topic “light source,” for instance. CLIP usually gives higher scores to electric lamps in wealthier homes compared to kerosene lamps in poorer ones.
Geographic bias
There’s also a geographic bias in CLIP, with most countries with low scores being from low-income African countries. This bias might lead to less diversity in big image datasets and make applications using CLIP not represent low-income, non-Western homes well.
“Many AI models aim to achieve a ‘general understanding’ by utilizing English data from Western countries. However, our research shows this approach results in a considerable performance gap across demographics,” the researchers explain.
“This gap is important in that demographic factors shape our identities and directly impact the model’s effectiveness in the real world. Neglecting these factors could exacerbate discrimination and poverty. Our research aims to bridge this gap and pave the way for more inclusive and reliable models.”
Making things better
The experts suggest a few practical steps for AI developers to make fairer AI models:
- Get datasets from different places to teach AI tools about a variety of backgrounds.
- Make sure the measurements used to judge AI work for everyone, taking into account where people live and how much money they make.
- Write down who’s in the datasets used to train AI models.
“The public should know what the AI was trained on so that they can make informed decisions when using a tool,” they conclude.

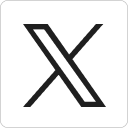



