Human pathologists are skilled at spotting when tissue samples from one patient accidentally appear on another patient’s microscope slides—a situation known as tissue contamination. However, artificial intelligence (AI) models, usually trained in controlled and flawless simulated environments, can struggle with this issue, as highlighted in a recent study from Northwestern Medicine.
“We train AIs to tell ‘A’ versus ‘B’ in a very clean, artificial environment, but, in real life, the AI will see a variety of materials that it hasn’t trained on. When it does, mistakes can happen,” the researchers explain.
False promises
“Our findings serve as a reminder that AI that works incredibly well in the lab may fall on its face in the real world. Patients should continue to expect that a human expert is the final decider on diagnoses made on biopsies and other tissue samples. Pathologists fear — and AI companies hope — that the computers are coming for our jobs. Not yet.”
The scientists taught three AI models to analyze microscope slides containing placenta tissue for (1) identifying blood vessel damage, (2) estimating gestational age, and (3) classifying macroscopic lesions. Additionally, a fourth AI model was trained to detect prostate cancer in tissues obtained from needle biopsies. To challenge these models, scientists exposed them to small sections of contaminant tissue (such as bladder or blood) randomly taken from other slides. Subsequently, the researchers assessed how the AIs responded.
The study revealed that all four AI models were overly focused on the contaminant tissue, leading to errors in diagnosing blood vessel damage, estimating gestational age, identifying lesions, and detecting prostate cancer.
Surprising findings
While tissue contamination is a familiar issue for pathologists, the study emphasizes that non-pathologist researchers or doctors may find it surprising. Pathologists, accustomed to examining 80 to 100 slides daily, typically encounter two to three slides with contaminants but are trained to disregard them.
When examining tissue on slides, human pathologists survey a limited field within the microscope before moving on to new fields. After reviewing the entire sample, they consolidate the gathered information for a diagnosis. Similarly, AI models operate comparably, but the study found that AI was prone to confusion when faced with contaminants.
“The AI model has to decide which pieces to pay attention to and which ones not to, and that’s zero sum,” the researchers explain. “If it’s paying attention to tissue contaminants, then it’s paying less attention to the tissue from the patient that is being examined. For a human, we’d call it a distraction, like a bright, shiny object.”
The AI models displayed a significant focus on contaminants, suggesting a challenge in encoding biological impurities. The study authors recommend that practitioners address and enhance the quantification of this issue.
While prior AI studies in pathology have explored various image artifacts like blurriness, debris on slides, folds, or bubbles, this research marks the first instance of investigating the impact of tissue contamination on AI models.

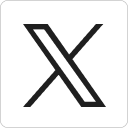



