In the ’80s, researchers tried a job-training program called JOBSTART in 13 U.S. cities. It didn’t do much in 12 places, but in San Jose, California, things looked up. After a few years, workers there were making about $6,500 more per year compared to those not in the program.
So, in the ’90s, the U.S. Department of Labor gave it a shot in 12 more cities. Turns out, lightning didn’t strike twice. San Jose stayed the success outlier.
Experts call this the “winner’s curse.” It’s like when you try something, and it works great, but the next time, not so much. It’s a problem for officials, company bosses, and scientists. Picking something that worked before might end up being a downer. What goes up often comes down, and that’s the challenge they face.
Avoiding the winner’s curse
Research from MIT explores the curse and how it can be overcome, with the researchers developing new tools that can help society to avoid it in the future.
The winner’s curse has been around in social science for a few decades. It’s not just a social thing; it pops up in the natural sciences too. The researchers mention that it shows up in genome-wide association studies, where they try to connect genes with traits.
When results that seem important don’t pan out, there could be different reasons. Sometimes, when people try to repeat experiments or programs, they don’t do everything the same way. Other times, things might just vary randomly, causing this kind of situation.
“Imagine a world where all these programs are exactly equally effective,” the researchers explain. “Well, by chance, one of them is going to look better, and you will tend to pick that one. What that means is you overestimated how effective it is, relative to the other options.”
Why it happens
Examining the data carefully can help figure out if the unusual result happened because the program genuinely worked differently or if it was just random ups and downs.
To tell the difference, the researchers came up with new ways to analyze the results. They introduced new “median unbiased” estimators, a way of predicting outcomes that are just as likely to be too high as they are too low, even in situations with the winner’s curse.
These methods also give confidence intervals, helping to measure how certain or uncertain the estimates are. The scholars also suggest “hybrid” approaches, blending various ways of looking at research data, which, as they demonstrate, often provide more accurate results than other methods.
Clearer boundaries
Using these new methods, the authors set clearer boundaries on using experimental data—like confidence intervals and unbiased estimates. To check if their approach works, they tested it on different social science research cases, starting with the JOBSTART experiment.
Interestingly, when it comes to experimental outcomes going off the usual path, the scholars found that the San Jose result from JOBSTART wasn’t likely just due to chance. The differences were big enough that there might have been changes in how the program was done or in its environment, compared to the other programs.
To put the hybrid inference method to the test, the researchers applied it to another research topic: programs providing housing vouchers to assist people in moving to neighborhoods with higher economic mobility.
Making progress
Previous nationwide economic studies showed that some areas offer better economic opportunities than others, even when everything else is equal. Building on these findings, the researchers collaborated with officials in King County, Washington, to create a program helping voucher recipients move to areas with more opportunities. However, predicting how well these programs would work might be tricky due to imperfect estimates of the opportunity levels in each neighborhood, potentially falling prey to the winner’s curse.
The authors then used the hybrid inference method to examine data at the neighborhood level in 50 “commuting zones” (basically, metro areas) across the U.S. This method once again helped them gauge the certainty of earlier estimates.
Initial estimates in this context suggested that children growing up in households at the 25th percentile of annual income in the U.S. would see a 12.25 percentage-point increase in adult income through housing relocation programs. The hybrid inference method proposed a slightly lower impact of 10.27 percentage points, still a meaningful improvement.
Small but meaningful
Indeed, as the authors write in the paper, “even this smaller estimate is economically large,” and “we conclude that targeting tracts based on estimated opportunity succeeds in selecting higher-opportunity tracts on average.” At the same time, the scholars saw that their method does make a difference.
Overall, the researchers say, “the ways we measure uncertainty can actually become themselves unreliable.” That problem is compounded, he notes, “when the data tells us very little, but we’re wrongly overconfident and think the data is telling us a lot. … Ideally you would like something that is both reliable and telling us as much as possible.”

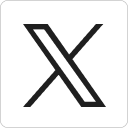



