With Microsoft and Google rolling out gen-AI supported assistants this year, the hope among the tech industry is clearly that these tools will turn us into smarter decision makers. Is that really the case, however? A few studies suggest that AI may actually make our decisions less effective.
For instance, research from Northwestern University looked at how the use of the AI-powered HawkEye system in tennis affected how officials made decisions about whether a ball was in or out.
Getting it right
The study found that the introduction of Hawk-Eye oversight has led human officials to reduce their errors by 8%. The most significant improvement was seen in multi-shot rallies following a successful serve and return. However, when the researchers focused on serves, particularly those where the ball landed within 20mm of a line, they observed a surprising increase in the error rate.
In these cases, umpires and line judges altered their strategy. Before Hawk-Eye, they were more likely to call a serve out when it was actually in. After Hawk-Eye, they became even more prone to allowing serves that were genuinely out. Post-Hawk-Eye, for every 100 mis-hit serves, umpires let 39 go unchallenged, compared to 26 in the earlier era.
This shift in behavior can be explained by the fact that overlooked faults are less disruptive in tennis than incorrect calls of “out” because the latter prematurely end the point. Additionally, incorrect calls can lead to dissent from both players and the crowd when the mistake is revealed on the big screen. It appears that human officials opt for the less reputationally risky choice, even if it results in more incorrect calls.
Life and death
Research from the University of Michigan performed a similar analysis in a slightly more important domain: healthcare. The researchers analyzed the use of AI software that was designed to provide an early warning for potential sepsis.
The tool, which was developed by the electronic medical record software company Epic, automatically generates a risk estimate for patients every 20 minutes. The aim is to allow doctors to spot the signs of sepsis before things get serious.
“Sepsis has all these vague symptoms, so when a patient shows up with an infection, it can be really hard to know who can be sent home with some antibiotics and who might need to stay in the intensive care unit. We still miss a lot of patients with sepsis,” the researchers explain.
A major killer
The researchers highlight that sepsis is a major problem in hospitals, and is responsible for around a third of all hospital-based deaths in the United States. As a result, catching it as early as possible is crucial to patient survival.
The study suggests, however, that AI systems aren’t really extracting any more useful information from our patient records than clinicians are.
“We suspect that some of the health data that the Epic Sepsis Model relies on encodes, perhaps unintentionally, clinician suspicion that the patient has sepsis,” the authors explain.
Effective warning
Patients may not undergo blood culture tests and antibiotic treatments until they show symptoms of sepsis. Although this data could enable AI to accurately identify sepsis risks, it might be added to medical records too late for clinicians to initiate early treatments.
This timing mismatch was evident in the assessment of the Epic Sepsis Model’s performance for 77,000 adults hospitalized at the University of Michigan Health. The AI had previously estimated each patient’s sepsis risk as part of standard operations, allowing researchers to analyze the data. Around 5% of patients had sepsis.
Evaluating the AI’s performance, the team calculated the probability that the AI assigned higher risk scores to patients later diagnosed with sepsis compared to those never diagnosed. When considering predictions made at all stages of the hospital stay, the AI correctly identified high-risk patients 87% of the time.
Falling accuracy
However, its accuracy dropped to 62% when using data recorded before patients met sepsis criteria. Notably, the model only assigned higher risk scores to 53% of patients who later developed sepsis when predictions were limited to before a blood culture was ordered.
These findings suggest that the model relied on whether patients received diagnostic tests or treatments when making predictions. However, at that point, clinicians already suspect sepsis, making the AI predictions less impactful.
“We need to consider when in the clinical workflow the model is being evaluated when deciding if it’s helpful to clinicians,” the researchers explain. “Evaluating the model with data collected after the clinician has already suspected sepsis onset can make the model’s performance appear strong, but this does not align with what would aid clinicians in practice.”
So while the tech companies are understandably bullish about AI’s prospects for improving our decision-making, the evidence to date suggests that the jury remains out.

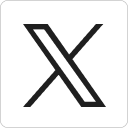



