New research from the University of Bath suggests that lenders in indirect retail channels, like car dealerships, could significantly boost their profits by incorporating artificial intelligence (AI) into their operations. By leveraging AI to support salespeople rather than relying solely on their discretion to price loans, these lenders could increase their profit margins by more than a third.
The study, which focused on lending practices in Canadian car dealerships, also highlighted the potential of AI and centralized pricing at company headquarters to address human bias. This approach could lead to improved access to loans for individuals with traditionally low credit approval rates. By avoiding suboptimal loan pricing decisions made by salespeople, AI could help prevent these individuals from being denied credit opportunities.
“Essentially we looked at whether analytics-based models were better at pricing loans for the average customer than salespeople and found that, as long as a company has access to rich data on their customers, AI models can pinpoint price sensitivity better than people can,” the researchers explain.
Tailored pricing
“Many companies have such data but are not making best use of it. But shifting to discriminatory—or tailored—pricing by AI has the potential to raise profits significantly. Equally importantly, it could open up lending to people who have struggled to get credit in the past because analytics-based approaches can pinpoint pricing that will work for them and also safeguard a balance of profit and risk for a lender.”
The study revealed that implementing analytics-driven pricing, which considers customer risk, and optimizing salespeople’s commissions could lead to a significant profit increase of 34%.
The researchers highlighted that discriminatory pricing practices, such as setting loan prices based on a customer’s credit score, may not be permissible in all countries. Many nations mandate that loans must be offered at the same price to all consumers, regardless of individual credit scores or other factors.
“Also, a lot of financial institutions have been wary of embracing AI and discriminatory pricing, possibly because of fears of a customer backlash over AI bias, which is a well-reported phenomenon,” the authors explain. “However, we would argue that using AI that is based on well understood and transparent machine learning, rather than salesforce pricing delegation, and ‘clean’ data that excludes demographics such as age, gender or race, has the potential to mitigate human and AI bias in such decisions.”
Mitigating bias
In a bid to mitigate bias, the study concentrated on various factors including consumer credit scores, loan-to-value ratios, vehicle types, and vehicle prices. Notably, it centered its analysis on an average customer, rather than individuals at the extreme ends of credit ratings.
Although the study primarily delved into the realm of car loans, the authors note that its findings could be applicable to any lending scenario involving assets, such as household appliances.
“However, deploying in sectors where personal relationships were key to transactions and pricing, such as business-to-business, would likely be of more limited benefit,” they conclude.

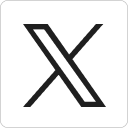



