A recent study by researchers from Stony Brook University, Stanford University, and the University of Pennsylvania used AI and social media to check for depression and anxiety in almost half of American counties. They found that the AI method was more reliable than traditional surveys.
Depression and anxiety are big issues in society. In 2019, about 10.8% of American adults had one of these conditions, which are linked to more suicides and opioid-related deaths.
A fresh approach
Normally, we ask people in expensive phone surveys if they feel sad or worried. But these surveys often don’t give enough detail about how mental health is changing locally.
So, the researchers tried something new: they looked at social media posts. They analyzed over a billion tweets from two million users in 1,418 US counties. This gave them a big dataset to understand mental health at the community level.
Their approach went beyond the usual surveys, tapping into the natural language people use online. This helps paint a clearer picture of mental health in different areas. Policymakers and mental health advocates can use this data to better target support where it’s needed most.
AI health assessments
The researchers found that Language-based mental health assessments (LBMHAs) were more dependable than the largest public opinion polls focused on public well-being. Moreover, LBMHAs demonstrated strong external validity, meaning they accurately predicted other community indicators linked to mental health, such as mortality rates, better than traditional surveys. Additionally, the AI-generated scores showed greater predictive power regarding various social, economic, and political factors.
The development of the LBMHAs system represents nearly a decade of meticulous work aimed at creating robust mental health evaluations. This process involved pinpointing the locations of Twitter/X users, analyzing their language usage patterns from tweets/X posts, aggregating these insights into regional assessments, and fine-tuning AI models to effectively analyze language for mental health estimation on Twitter/X.
Remarkably, this approach yielded depression rates correlating closely with expressions of sadness and anxiety rates aligning with indications of worry, as collected through representative phone surveys in 2020.
“The main result of this study was a comparison of how well our AI model’s predictions lined up with survey-based methods and how computational methods enable new resolutions of mental health studies that were previously not possible,” the researchers say.
Better results
The study revealed that the proposed system surpassed survey methods by a notable margin of 10 percentage points when correlating with external factors such as education, housing, income, and social interaction.
Acknowledging the challenges inherent in capturing signs of mental health issues through language patterns and online personas, the researchers underscored the complexities involved in interpreting such signals within social media contexts.
“Social media measures allow us to track depression and anxiety—in principle—in real-time. Social media platforms are constantly changing in their leadership, policies, and how researchers can access data for the common social good,” they explain.
The authors suggest that public health authorities integrate language-based assessments into their existing survey data to gain a more immediate and nuanced understanding of community health trends.
They highlight the advantage of incorporating observed, rather than self-reported, psychological states, which helps circumvent the tendency of individuals to downplay or conceal less socially acceptable or stigmatized traits, including the presence of mental health conditions.

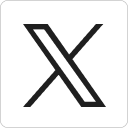



