Predictive algorithms commonly used by colleges and universities to gauge student success may exhibit racial bias against Black and Hispanic students, according to research from the University of Texas at Austin.
The study found that these models also tend to overestimate the potential success of white and Asian students.
Biased results
“Our results show that predictive models yield less accurate results for Black and Hispanic students, systematically making more errors,” the researchers said.
The study revealed that these models incorrectly predict failure for Black and Hispanic students 19% and 21% of the time, respectively, compared to false negative rates for white and Asian students of 12% and 6%. Conversely, the models incorrectly predict success for white and Asian students 65% and 73% of the time, respectively, compared to false positive rates for Black and Hispanic students of 33% and 28%.
“Our findings reveal a troubling pattern—models that use common features to predict college student success often forecast worse outcomes for racially minoritized groups and are frequently inaccurate,” the authors explained. “This underscores the need to address inherent biases in predictive analytics in educational settings.”
The study used nationally representative data spanning 10 years from the U.S. Department of Education’s National Center for Education Statistics, including 15,244 students.
The study also highlights the potential value of using statistical techniques to reduce bias, though limitations remain.

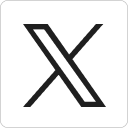



