A growing number of people are using the internet to find a partner, but can online dating provide insights into how we perceive other forms of artificial intelligence? After all, finding a mate is one of the most important things in our life, and many of us are relying on AI to pair us up with suitable people according to our desires, our interests, and even our relative attractiveness.
While data scientists can create AI models to predict complex outcomes like a couple’s chances of a second date, will users trust AI recommendations or prefer their own judgments? A recent Wharton study used the context of predicting speed dating outcomes to explore what influences trust in AI. The study is driven by research showing that, despite the high performance of AI systems, users often hesitate to trust them.
“Yet, despite the high performance of these systems, users have not readily adopted them,” the researchers explain. “This phenomenon is not a new one, as users’ reluctance to adopt algorithms into their decision making has been demonstrated over time.”
Building trust
Trust in technology typically emerges based on either the performance of the technology or our understanding of how the technology arrived at its decision. For instance, even if the AI-based decision is fairly sound, if users don’t understand how the decision was made, it may limit the trust they have in the system.
“Users may not trust systems whose decision processes they do not understand,” the authors explain. “We investigate this proposition with a novel experiment in which we use an interactive prediction task to analyze the impact of interpretability and outcome feedback on trust in AI and on human performance in AI-assisted prediction tasks.”
The study’s findings challenge the common belief that users will trust AI more if they understand how a model arrived at its prediction—known as interpretability. Instead, a bigger driver of trust was outcome feedback on whether the AI’s predictions were correct or not.
Growing over time
Participants tended to build trust over time based on whether following the AI improved or worsened their performance on recent predictions. The paper is one of the first to compare interpretability and outcome feedback to understand how they impact the development of trust in AI, and therefore, on user performance.
Interestingly though, the study found that neither the performance of the AI nor its explainability were that important in terms of supporting the development of trust, which highlights the challenges involved for developers.
“Augmenting human performance via AI systems may not be a simple matter of increasing trust in AI, as increased trust is not always associated with equally sizable improvements in performance,” the authors explain.
Losing faith
Research from the University of Michigan shows how quickly faith in technology can falter. The study found that humans are less forgiving of robots after they make a series of errors, and that regaining their trust again is extremely difficult.
Just like human coworkers, robots can make mistakes that erode trust. The study explored four strategies to restore trust, including apologies, denials, explanations, and promises of trustworthiness.
The experiment involved 240 participants working with a robot colleague on a task, with the robot occasionally making errors and then offering a repair strategy. The results showed that after three mistakes, none of the repair strategies were able to fully restore trust.
“By the third violation, strategies used by the robot to fully repair the mistrust never materialized,” the researchers explain.
The importance of trust
Trust plays a key role in our daily lives and especially in the relationships we have, whether with other individuals, organizations, or even technology. However, businesses face significant challenges in designing, managing, and measuring trust in digital technology. This inadequacy in “trust literacy” causes organizations, especially those in data-intensive environments, to hesitate or refrain from adopting new digital technologies, risking their growth and competitiveness.
Of course, while AI interpretability did not significantly impact trust, it did have other uses, such as helping developers debug models or meeting legal requirements around explainability. The Wharton findings could encourage further research on improvements in AI interpretations and new user interfaces to better impact trust and performance in practice.
As customers hand over private data, make online decisions on products, or engage with sophisticated technologies like autonomous systems and facial recognition payments, trust is crucial if employees and customers are to engage with AI to a full extent. Figuring out the best way to gain and maintain that trust is going to be crucial to the success of AI systems now and in the future.

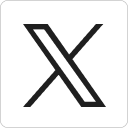



