New research indicates that using AI in mortgage lending could lead to discrimination against Black applicants, but a surprisingly simple fix may mitigate this bias.
In an experiment, Lehigh University researchers found that leading commercial large language models (LLMs) consistently recommended denying more loans and charging higher interest rates to Black applicants compared to otherwise identical white applicants. This discovery is particularly concerning given the long-standing racial disparities in homeownership.
Historical biases
“These findings suggest that LLMs are learning from the historical data they are trained on, which likely includes a legacy of racial disparities in mortgage lending, and may be incorporating racial bias triggers from other contexts,” the researchers explain.
The study used real mortgage application data from the 2022 Home Mortgage Disclosure Act (HMDA) dataset, creating 6,000 experimental loan applications by manipulating race and credit score variables. The results were striking: Black applicants faced higher barriers to homeownership, even when their financial profiles were the same as white applicants.
When using OpenAI’s GPT-4 Turbo LLM, the study found that Black applicants would need credit scores about 120 points higher than white applicants to achieve the same approval rate and around 30 points higher to secure the same interest rate. Hispanic applicants also faced bias, though generally to a lesser extent.
Bias was most pronounced in “riskier” applications—those with low credit scores, high debt-to-income ratios, or high loan-to-value ratios.
Consistent biases
The researchers tested various LLMs, including OpenAI’s GPT 3.5 Turbo and GPT 4, Anthropic’s Claude models, and Meta’s Llama models. While bias in interest rate recommendations was consistent across models, approval rates varied significantly. ChatGPT 3.5 Turbo showed the highest level of discrimination, whereas ChatGPT 4 (2023) exhibited almost none.
“It’s surprising to see racial bias, given the significant efforts by LLM creators to reduce bias and the strict regulations on fair lending,” the researchers note. They point out that the models’ training data likely includes federal regulations prohibiting the use of race in lending decisions.
However, the study revealed an unexpected solution: instructing the LLMs to avoid bias in their decisions. When explicitly told to ignore race, the racial bias nearly disappeared.
“It didn’t just reduce the bias; it almost completely eliminated it,” the authors conclude.

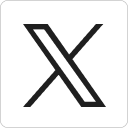



