That AI suffers from biases is well documented. The scale of those biases is reflected in a couple of studies from the University of Michigan, which show that Black patients are less likely to receive the kind of medical tests that could detect severe diseases than their white peers.
This then means that Black patients are more likely to be assumed to be healthy, even when they’re not, with this mistaken data being used to train AI systems and therefore perpetuate the situation and underestimate illness in Black patients. Thankfully, the second study showcases how this situation can be rectified.
Unequal testing
The researchers found that medical testing rates are nearly 5% higher for white patients than for Black patients, even when all other factors are identical. They suggest that this might be partly explained by the different hospital admission rates, with white patients more likely to be assessed as ill, and therefore admitted to hospital.
“If there are subgroups of patients who are systematically undertested, then you are baking this bias into your model,” the researchers explain. “Adjusting for such confounding factors is a standard statistical technique, but it’s typically not done prior to training AI models. When training AI, it’s really important to acknowledge flaws in the available data and think about their downstream implications.”
To make AI accurately predict illnesses across different groups of patients, researchers need to account for biases in medical data. One approach is to train the AI on less biased data, like records of patients who have had diagnostic tests. But this can create its own problems, since it could make the model less accurate for healthier patients who don’t get tested as often.
Fixing the bias
To fix the bias without leaving out patient records, researchers designed an algorithm that estimates whether untested patients might be ill, based on vital signs like blood pressure and demographic factors like race. The algorithm considers race because records of Black patients are often more affected by testing bias.
The researchers tested the algorithm on simulated data, introducing a known bias by relabeling some diagnosed patients as “untested and healthy.” Using this biased data to train a standard machine-learning model, they found that the algorithm-corrected model could identify sepsis about 60% of the time—much better than random guessing, which happened without the algorithm.
The model’s accuracy was close to that of a model trained on an ideal, unbiased dataset where everyone is equally tested. While such datasets are rare in the real world, the researchers’ method allowed the AI to work almost as well as it would with unbiased data.
“Approaches that account for systematic bias in data are an important step towards correcting some inequities in healthcare delivery, especially as more clinics turn toward AI-based solutions,” the authors conclude.

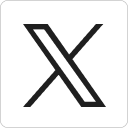



