Researchers at the University of Michigan have found a way to make training large language models, like GPT, 30% more energy-efficient without slowing down the process. This technique could save enough power to supply 1.1 million U.S. homes by 2026, according to projections from Wells Fargo. It could also help reduce the growing carbon emissions from data centers, which, by the International Monetary Fund’s estimate, could reach 1.2% of the global total by 2027.
Some experts argue that AI’s environmental costs are justified, as it can help fight climate change by optimizing supply chains, managing the energy grid, and advancing research. But these benefits don’t excuse wasting energy. In fact, much of the power used in training models doesn’t speed up the process or improve accuracy.
A wasteful process
The waste occurs because training jobs must be split across many specialized processors, or GPUs, to handle large amounts of data. These tasks are hard to divide evenly because some need to stay together on the same processor, like keeping a book series on one shelf. This imbalance often means some processors have far more work than others, creating inefficiency.
Right now, every processor runs at full speed, even if some finish early, wasting energy while they wait for others to catch up. The researchers tackled this with a tool called Perseus, which identifies the “critical path,” or the tasks that take the longest, and slows down other processors so they all finish around the same time, cutting energy use.
The researchers say this could make AI more accessible to places with limited power. Without such tools, regions might be forced to use less accurate models or rely on distant services, which could widen gaps in technology access.
Perseus, now available as open-source software through the AI energy tool Zeus, was tested on GPT-3 and several other models. It points to a more energy-efficient path forward for AI.

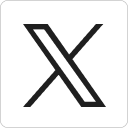



