Recently I wrote about an interesting new project that’s helping to deepen our understanding of the cosmos. A team from the Department of Energy’s Lawrence Berkeley National Laboratory (Berkeley Lab) are working on a prototype of an army of 5,000 robots that can head out into the galaxy.
The machines, which are known as ProtoDESI, come in ‘platoons’ of 10 robots and are designed to help us enhance the accuracy of the Dark Energy Spectroscopic Instrument (DESI). DESI is intended to provide a 3D map of the universe and allow scientists to further explore things like dark matter.
Machine learning
A second team, from University College London, are using machine learning techniques in their search for habitable worlds. The team have developed a deep belief neural network, which they’ve called RobERt (Robotic Exoplanet Recognition. The work, which is documented in a recently published paper, aims to detect light emanating from distant worlds and sift out useful information from that data.
“Different types of molecules absorb and emit light at specific wavelengths, embedding a unique pattern of lines within the electromagnetic spectrum,” the researchers say. “We can take light that has been filtered through an exoplanet’s atmosphere or reflected from its cloud-tops, split it like a rainbow and then pick out the ‘fingerprint’ of features associated with the different molecules or gases. Human brains are really good at finding these patterns in spectra and label them from experience, but it’s a really time consuming job and there will be huge amounts of data.”
RobERt is designed to learn from the examples it’s fed with and thus build a degree of expertise of it’s own so that it can develop a feel for which bits of data are most promising for further analysis. The benefit of automating this is that it rapidly speeds up the process, with what might normally take many days taking a few seconds.
RobERt was put through its paces with over 85,000 simulated spectra that included a number of different types of exoplanet. The rate of learning by the system was tested at various intervals alongside a control spectra. By the end of the process, RobERt was capable of recognizing spectra with an accuracy of 99.7%.
“RobERt has learned to take into account factors such as noise, restricted wavelength ranges and mixtures of gases,” the team say. “He can pick out components such as water and methane in a mixed atmosphere with a high probability, even when the input comes from the limited wavebands that most space instruments provide and when it contains overlapping features.”
Data analysis
Not only can the system analyze data that is fed into the system, it can also act in a kind of dream state whereby it can generate it’s own full spectra from it’s experiences.
“Robots really do dream. We can ask RobERt to dream up what he thinks a water spectrum will look like, and he’s proved very accurate,” the team reveal. “This dreaming ability has been very useful when trying to identify features in incomplete data. RobERt can use his dream state to fill in the gaps. The James Webb Space Telescope, due for launch in 2018, will tell as more about the atmospheres of exoplanets, and new facilities like Twinkle or ARIEL will be coming online over the next decade that are specifically tailored to characterising the atmospheres of exoplanets. The amount of data these missions will provide will be breathtaking. RobERt will play an invaluable role in helping us to analyse data from these missions and find out what these distant worlds are really like.”
With data such a huge part of astronomy, it’s to be expected that automation will play a bigger role in our exploration of the cosmos. These two projects are interesting examples of our direction of travel.

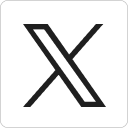



