I’ve written a few times recently about a number of projects that aim to improve the ability of artificial intelligence to see, and importantly, comprehend the world in the same way humans do.
One of these was a fascinating project from a team at the Dyson Robotics Lab at Imperial College London is very interesting. The team developed some open source software, which they’re calling ElasticFusion, which gives robots a better understanding of their environment, and their place in it.
The ultimate aim is to allow robots to operate more safely in the home by mapping the environment and identifying elements within it.
A recent study highlights a similar project from a team from Northwestern University. The team have developed a computational model that aims to perform at human levels in various intelligence tests.
“The model performs in the 75th percentile for American adults, making it better than average,” the team say. “The problems that are hard for people are also hard for the model, providing additional evidence that its operation is capturing some important properties of human cognition.”
Building on CogSketch
The model is based upon CogSketch, which is an AI platform previously built by the lab. It was developed to help AI solve visual problems and understand basic drawings. This is one of the hallmarks of human intelligence, but has traditionally been difficult for machines to master. The researchers believe that their work provides another milestone in shrinking the gap between human and computer cognition.
The model was tested out on Raven’s Progressive Matrices, a nonverbal test that is commonly used in education to test for abstract reasoning capabilities. The system managed to perform better than the average American in the test.
“The Raven’s test is the best existing predictor of what psychologists call ‘fluid intelligence, or the general ability to think abstractly, reason, identify patterns, solve problems, and discern relationships,'” the researchers say. “Our results suggest that the ability to flexibly use relational representations, comparing and reinterpreting them, is important for fluid intelligence.”
The model was able to both understand and then utilize sophisticated relational representations, which the researchers believe is a key step towards higher-order cognition. It’s crucial for understanding things such as analogies and moral dilemmas, which form such an integral part of human behavior and problem solving.
“Most artificial intelligence research today concerning vision focuses on recognition, or labeling what is in a scene rather than reasoning about it,” the team conclude. “But recognition is only useful if it supports subsequent reasoning. Our research provides an important step toward understanding visual reasoning more broadly.”

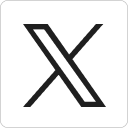



