Whenever something serious happens, we usually try and determine cause and effect. What was it that caused this thing to unfold the way it did? Whilst the theory is nice, often we employ some rather dubious explanations to try and explain the series of events. Superstitions perhaps, or correlation rather than causation.
There have been attempts in the past to generate mathematical models for general causality, but they haven’t been particularly effective, especially for more complex problems. A new study from the University of Johannesburg, South Africa and National Institute of Technology Rourkela, India, has attempted to use AI to do a better job.
“Uniquely, the model can identify multiple, hierarchical causal factors. It works even if data with time sequencing is not available. The model creates significant opportunities to analyse complex phenomena in areas such as economics, disease outbreaks, climate change and conservation,” the researchers say. “The model is especially useful at regional, national or global level where no controlled or natural experiments are possible.”
Finding causality
The team hope that their work can help to answer some of the trickiest problems facing society today. Why are certain areas suffering from poverty? Why is obesity or diabetes higher among certain groups? Why does the gender pay gap exist?
They believe that their approach helps to identify the multiple driving factors causing an issue to occur. They’re things that the team refer to as independent parent causal connections.
“You can also see which causal connections are more dominant than the others. With a second pass through the data, you can also see the minor driving factors, what we call the independent child causal connections. In this way, it is possible to identify a possible hierarchy of causal connections,” they explain.
They are confident that their Multivariate Additive Noise Model (MANM) provides a significantly better causal analysis than current models when using real-world datasets.
It aims to understand not just the patterns visible in the data, but why those patterns exist. By extending significantly beyond the two causal factors that most models use, they believe they have a reliable way of doing that.
“MANM is based on Directed Acyclic Graphs (DAGs), which can identify a multi-nodal causal structure. MANM can estimate every possible causal direction in complex feature sets, with no missing or wrong directions,” the team explain.
By examining a much wider range of contributing factors, the team are confident that their approach can prove extremely effective in uncovering the underlying causes behind a range of thorny problems. Time will tell just how well placed their confidence proves to be.

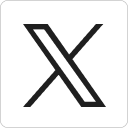



