The interest in the impact of new technologies on the labor market shows little signs of abating, with continuing uncertainty about the scale and nature of the impact. The latest attempt to shed light on the matter comes via a recently published study from the MIT Media Lab.
The authors acknowledge the historical resilience seen in the labor market, as past generations have successfully navigated the rise of new, more powerful technologies. They aim to understand the roots of this resilience to enable a better exploration of whether the AI-driven technologies of today pose a more existential threat than past technologies.
The inherent lack of clarity surrounding the debate, and in particular around the kind of skills and tasks that make up work today, has resulted in a number of different perspectives existing concurrently. The authors propose a number of solutions for improving data on the skills required in the workforce today, and from that the potential for AI to automate or augment those skills.
Lack of skills data
The first challenge proposed by the team revolves around a fundamental lack of available skills data. Traditional labor data utilizes aggregate statistics, which can render it difficult to appreciate the nuances inherent in jobs today. For instance, a junior consultant will perform very different roles to a senior consultant, yet their labor market classification is often identical.
The U.S. Department of Labor’s O*NET database has seen consistent use in various other attempts to model the potential impact of AI on the labor market, but whilst O*NET is updated twice per year and is therefore a significant improvement on previous datasets, it is still unlikely to be keeping pace with the changes in the workforce. To compound this, studies such as the famous Frey and Osborne study in 2013 have aggregated skills rather than focusing on a more granular exploration.
“For instance, according to O*NET, the skill “Installation” is equally important to both Computer Programmers and to Plumbers, but, undoubtedly, workers of these occupations are performing very dissimilar tasks when they are installing things on the job,” the authors explain.
Improving our ability to project possible impacts will require a much better understanding of the dynamics of specific skills, whilst appreciating the income of occupations requiring those skills can allow a rudimentary valuation of those skills to be made. The authors suggest some of the online work platforms might offer insight into the skills demanded in the workplace today, with job postings capable of capturing the fluctuation in demand for specific skills. Of course, access to this data is currently restricted, but the authors believe a secure environment for hosting such data could overcome any privacy and competitiveness concerns and allow research to be more informed as a result.
Limited understanding of resilience
A second significant barrier is a detailed understanding of the resilience, both of individuals and of communities to technological disruption. Numerous people have argued that AI is such a different technology to any we’ve seen previously that trying to use historical data to extrapolate impact is foolhardy. This creates an inherently uncertain situation as past data cannot be used to construct policies about the future.
A well trodden example is the introduction of ATM machines into banks, which were predicted to cause a reduction in demand for bank tellers, yet the reverse has actually happened, with the elasticity of demand for such roles largely the cause. The example illustrates the need to capture both the granular workplace skills and the macroscopic labor trends.
A good first step to address this challenge is to better map skill inter-dependencies so that training programs can be produced, both in terms of the curriculum and the location, to help people adapt to the technological landscape. Network science could help to better map these inter-dependencies, and therefore shed light on the impact of AI on the workforce.
“Mapping skill dependencies will require appropriate data handling methods,” the authors explain. “The ideal skills data should reflect the dynamic nature of skill representation, and so, the methods we use to detect, categorize, and measure the demand for skills must be adaptable as well.”
With so much data required, this is ironically an area that the authors believe AI can play a part, with machine learning capable of inferring patterns from large and complex data sets.
A lack of geographic complexity
A third barrier is the difficulty we have in appreciating the nuance of technological disruption by place. As with any technology, the impact of AI is likely to be felt at different levels in different places, yet many examinations of the topic to date have taken a national rather than regional perspective. Whilst the national picture may lead us in one direction, regional stories may differ significantly. Indeed, this has certainly been evident with historical disruptions to the labor market, either as a result of economic or technological change.
Part of the solution to this problem is to get better quality skills data, but it would also help to get fresh insight into the various mechanisms that underpin geographic trends today. This is especially so in a world in which labor mobility is still widely seen as a positive thing for a thriving economy. Therefore, it’s vital that we understand the skill sets in cities so that we can then better understand the mobility of workers.
“Artificial intelligence has the potential to reshape skill demands, career opportunities, and the distribution of workers among industries and occupations in the U.S., and in other developed and developing countries,” the researchers conclude. “Yet, researchers and policy makers are under-equipped to forecast the labor trends resulting from specific cognitive technologies, such as AI. Typically, technology is designed to perform a specific task which alters demand for specific workplace skills.”
The paper is interesting because it deliberately avoids making wholesale, yet incomplete, predictions about the number of jobs that may be affected, and focuses instead on the paucity of data that underpins many of these predictions, and the work needed to make such predictions more robust. Time will tell whether their advice is heeded.

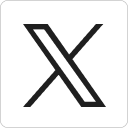



