Welfare dependency is a challenge for most governments around the world and researchers from the University of Exeter believe that AI can help. In a new study, they suggest that AI is around 20% more accurate at predicting the people who will go on to become long-term recipients of benefits than other methods.
It’s an approach the researchers believe could save governments billions by allowing earlier interventions to be made. The findings emerged after an analysis of people enrolled in the Australian social security system from 2014 to 2018.
This data included both socio-economic and demographic data for anyone who had received a form of welfare payment from the Centrelink social security system. This includes those claiming support for disability, unemployment, having children, or being a carer.
Welfare dependency
The researchers fed the algorithm a sample amount to 1% of this group, all of whom had registered in the system in 2014. The researchers then tracked the group over the following three years to see if they continued to receive state support.
“We found that the size and richness of the dataset on social security enrolees makes it ideal for a machine learning application, allowing the algorithms to achieve high performance by detecting subtle patterns in the data and by identifying new powerful predictors,” the researchers explain.
They then compared current methods of predicting welfare dependency with that of the algorithm. The current method uses a range of profiling indicators, such as age, sex, and education. The results reveal that the AI algorithm was around 22% more effective at predicting future income support than the existing methods.
This, the authors claim could save the Australian state around $1bn AUS in welfare payments, which equates to around 10% of all expenditure each year on unemployment benefits.
A new approach
The boost in accuracy is largely explained by the greater range of predictive factors that the AI system can consider compared to existing methods, with the AI-based tool factoring in around 1,800 factors in total. It’s a technology that the researchers believe could augment existing workers nicely.
“We do not believe algorithms should replace human expertise but rather act as its complement,” they explain. “Caseworkers could focus their attention and time providing a personalized service and targeting the appropriate support to individuals that the algorithm identifies as most at risk.”
For government programs to work, caseworkers need to know the individuals that are most at risk so that interventions can be targeted effectively. It’s a process that the AI-based system can support with by providing an effective and low-cost means of improving predictions without succumbing to any of the conscious and unconscious biases that human decision-makers succumb to.
“Governments are increasingly using machine learning to tackle social problems and to make resource-allocation decisions,” the researchers conclude. “For example, it has been used to help judges to improve bail-granting decisions, schools to identify students at risk of dropping out, and surgeons to screen patients for hip-replacement surgery.”

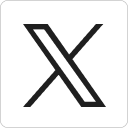



