Few people like to think of themselves as followers, but when it comes to evaluating products, services, or even colleagues, many of us end up following the crowd. This matters because group evaluations—whether it’s a restaurant’s Yelp ratings or performance reviews at work—can significantly influence the success of a product, service, or someone’s career.
New research from Yale shows how the design of evaluation processes can affect outcomes, demonstrating that people who submit ratings are often influenced by the scores they’ve already seen.
These findings are especially relevant in settings where livelihoods are on the line, such as gig platforms like Upwork or Uber, where ratings can make or break a worker’s career.
Previous history
The researchers wanted to understand how seeing previous ratings affects the ones that follow, essentially exploring what happens when the audience also becomes the evaluator. They studied data from an online platform where investment professionals—mostly from hedge funds and mutual funds—share and evaluate investment recommendations.
These professionals also rate the quality of each other’s recommendations. The study compared ratings from those who had seen prior scores with those made by people who hadn’t. Importantly, all the evaluators had some expertise, as their jobs involved evaluating investments.
Several theories suggest why evaluations might start to look alike: competition, where people follow peers to avoid falling behind; reputation management, where people align with others to signal agreement; and peer deference, where an evaluator assumes their peer knows better. Since the platform’s users were anonymous, the study could rule out reputation management as a factor, focusing on the other possibilities.
Rated users
The platform allowed users to rate recommendations on a scale of 1 to 5 in two areas: the quality of the investment analysis (the justification rating) and its expected stock performance (the return rating). The first four ratings were hidden from other users, but after the fourth, the average score became public.
The researchers found that when a rating was visible, professionals were less likely to submit their own. Without a public score, 10% of viewers submitted a rating; with a score visible, only 4% did.
Private ratings, hidden from other users, tended to vary. But once a rating became public, later evaluations quickly converged, aligning 54% to 63% more closely with the initial average. This shift was immediate, not gradual.
This pattern is striking because the evaluators were professionals with expertise in investment, and they were anonymous, meaning peer pressure should have been low. Yet, just seeing others’ ratings made them more likely to go along with the crowd.
Following the herd
Could it be that the converging ratings reflected a genuine consensus about quality? The data says no. The initial private ratings did not predict the future performance of the stocks, showing that higher ratings weren’t necessarily more accurate.
One thing that did reduce the herd effect was subject-area expertise. Professionals with specific experience in the industry being rated, like healthcare experts evaluating a pharmaceutical stock, were more likely to give ratings that differed from earlier ones.
These insights suggest ways to improve evaluation processes to get more accurate results. For instance, platforms could wait to show average ratings until more people have submitted their private ratings. Companies might also get better results by having experts weigh in before revealing collective feedback.
As companies increasingly involve employees in decision-making, these findings highlight the need to carefully design evaluation processes. Without careful planning, these processes could lead to biased decisions rather than fair ones.

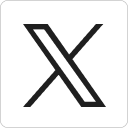



