It’s fair to say that we’re still in the experimental phase when it comes to generative AI in the workplace, which has meant that few businesses have seen tangible gains from the technology to date.
Researchers from the London School of Economics found that around two-thirds of firms had begun using new digital technologies since the Covid pandemic, with many of these accelerating the pace of adoption since Covid hit. The energy crisis invoked by the invasion of Ukraine had no such impact on adoption.
The investments in technology were especially high in areas like sales and marketing, while companies also invested heavily in remote work platforms and the kind of cloud and cybersecurity technologies that underpin remote work. Interestingly, however, investments in AI were relatively modest, with around 25% of companies investing in this area, with another quarter planning to do so.
How are firms using AI?
The researchers looked at how firms are using AI and found that usage is highest in sales and marketing, with around a third of marketers using it, if only in an experimental way, and a further 20% planning to do so. Just 20% said they were using AI in core business functions, such as engineering, production, or management, with even less usage in areas like logistics and facilities management.
Most respondents said that they were using AI not to replace workers but to do things in a new or improved way. These gains have largely not materialized, however, with around 10% of firms reporting any significant impact from their investments to date. This hasn’t dented optimism, however, with around 40% of businesses expecting AI to deliver improvements in profitability and resilience in the coming years.
As organizations, and indeed employees, find their feet, here are a few possible use cases that can help to guide experiments. Let’s look back at previous generations of technology. They have tended to have a disproportionate impact on lower-ranked individuals, who were often unable to fully utilize the technology but could have gained significantly if they could do so. This is something we’ve seen with generative AI, as skilled professionals are less susceptible to their work being automated, while lower ranked individuals are also less able to capitalize on the technology as they lack the ability to craft the right prompts or dissect the responses they get.
Harnessing the power
The early stages of the genAI “goldrush” have seen the likes of Nvidia reap huge rewards from the enormous investment model makers are making into hardware. We are likely to see a more realistic wave of opportunities emerge as the dust settles, however, as individuals and organizations begin to creatively harness the capabilities genAI provides. This will be especially so for those able to craft new business models based on the technology.
In an ideal world, these business models should raise the tide for all boats, as a feature of generative AI is that it operates via a natural language interface and doesn’t require a great deal of technical knowledge to operate. Obviously that doesn’t neglect the importance of using the right prompts, which shouldn’t be underestimated, but the potential exists to improve the capabilities of lower-skilled workers as the barriers to entry should be lower.
For instance, customer service representatives could see a number of benefits from using generative AI, not least in using it to both log and analyze chat transcripts, which can often contain thousands of words of text. If agents can receive an almost instantaneous AI summary, it can save minutes per call for both the agent and the company.
Barriers to adoption
While it’s perhaps fair to say that a lack of clear use case has undermined the widespread adoption of generativeAI thus far, the LSE researchers found that financial constraints are a bigger barrier going forward.
This is followed by a need for more information, skill shortages, and uncertainties related to both policy and technology. Interestingly, these challenges extend to AI adoption as well, though with some notable differences. While information deficits remain a major obstacle for AI, financial concerns appear to be less significant—perhaps a reflection of the growing availability of affordable AI-enabled tools in recent years.
Looking ahead, the findings suggest a degree of optimism about AI’s future impact. Although the barriers to adopting digital and AI technologies are consistent with previous technologies, it is clear that information gaps pose a greater challenge for AI. This is likely due to its rapid development and the fact that its applications are not yet fully understood. These gaps will inevitably be filled as familiarity with the technology, in terms of both its capabilities and limitations, increases. Until that time, we should carry on exploring and experimenting to discover what works for us.

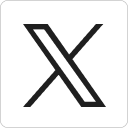



