Despite the tremendous improvements already made in driverless technologies, researchers continue to seek smarter cars. For instance, I wrote last year about a study to try and enable the car to predict the actions of other road users, such as cyclists and pedestrians.
Of course, this kind of sensual awareness is often quite expensive. I wrote recently about an approach by researchers at Cambridge University. The open source project, called Segnet, aims to make it more cost effective to scan an environment.
Whilst early signs are positive, the project is not yet strong enough to support driverless technology. A team from Stanford believe they may have an approach that is however.
Crowdsourcing awareness
The researchers wanted to build a huge database of fully annotated references that could be used to train machines to perform some of the more complex tasks that human drivers perhaps take for granted.
The approach, which is documented in a recent paper, sees participants play a driving game. The game runs off of a database of road conditions that was obtained by driving a real car around California to collect things like GPS data, laser scanning data and visual data.
This data is then fed into the virtual 3D environment. Players then help the AI behind the game, called Driverseat, to evaluate and understand this environment in a range of driving conditions.
The first forays down this path involved the relatively simple task of lane identification. This is something that we find quite straight forward, but it remains challenging for machines, especially in different light and weather conditions.
Gaming improvements
The players are presented with the same 3D environment the machine will ‘see’. This depiction also includes the first attempt made by the machine to identify the lanes.
The players are then tasked with correcting any errors encountered, with this then fed back into the AI to improve its knowledge.
The initial results are certainly interesting. The system was put through its paces on a part of the database that had thus far not been seen. It proved to be effective at identifying lanes in difficult circumstances, such as when the road curved, and even when vision was obstructed by other vehicles.
It proved less effective however at spotting off and on ramps, and was poor when visibility dropped as a result of shadow or road color changes.
Equally, it emerged that the system performed poorly when the sun was close to the horizon, with the authors believing this was largely due to the fact that Californian highways run north to south rather than east to west, thus giving the system less data about driving into a sunset or sunrise.
Next steps
Suffice to say, these issues are all things the team hope to work on in future, so it’s important at this stage to know what the system can’t do as much as what it can do.
The crowdteaching method however is certainly an interesting one, and one that has been used before to help improve artificial intelligence.
“We have shown how we can integrate people’s knowledge and experience on the roads to ‘teach’ machines to drive,” the team say.
It’s possible, therefore, that humans will play a role in the next generation of cars as our own knowledge begins to blend with that of the computers powering the cars.
It’s also likely that the crowdteaching method will be used to help machines learn a wide range of tasks that humans often take for granted.
It’s a project that’s well worth keeping an eye on.

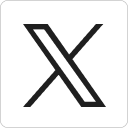



