The last year has seen some huge leaps in machine learning, such that they are now capable of detecting things such as emotions from a picture. They have also developed the ability to teach one another by following what is happening in videos.
Whilst they are capable of replicating what they see, it’s doubtful that machines really understand what they’re watching.
I wrote recently about an interesting project by researchers at Imperial College London, who developed some open source software, which they’re calling ElasticFusion, which gives robots a better understanding of their environment, and their place in it.
Automating understanding
A new project, called the Visual Genome, is aiming to provide a hub for understanding how machines are able (or not) to understand the world they operate in.
The platform was developed by professors at the Stanford Artificial Intelligence Lab and aims to tackle some of the toughest questions in computer vision, with the eventual goal of developing machines that can understand what it sees.
The project evolved out of ImageNet, which is a database of over a million images that are tagged according to their content. This would provide a testing ground for computers to see how well they could recognize the objects.
Visual Genome has then seen a richer level of meta information added to each image, with not only biographical information about the image, but the relationship it has with other objects and a description of what’s happening in the image.
Learning machines
This rich meta information was achieved using crowdsourcing, and the team hope to put machines to the test on the database sometime next year.
“You’re sitting in an office, but what’s the layout, who’s the person, what is he doing, what are the objects around, what event is happening?” the researchers say. “We are also bridging [this understanding] to language, because the way to communicate is not by assigning numbers to pixels—you need to connect perception and cognition to language.”
Ever more advanced deep learning will enable machines to process and understand these sort of complex scenarios, with significant implications for things such as driverless cars.
This is something a team from Cambridge University are attempting to tackle. Their work allows driverless cars to both identify their location in the absence of GPS, and also to identify other elements of the surrounding environment, all in real-time.
What’s more, the technology only requires a regular camera or smartphone to function, thus cutting huge sums from the cost of achieving such outcomes.
This highlights the range of approaches to understanding complex visual information automatically. Alongside these academic projects are efforts by tech giants such as Google, Facebook and Microsoft.
There is still a lot of work to do however before machines can begin to replicate our own ability to process visual information quickly and accurately.
It’s likely that researchers will need to lean on biology to achieve this, as our own efforts draw as much on common sense and memories as they do language and speech.
At the moment it seems as though each step forward presents fresh questions to be answered, but it’s certainly a fascinating area to monitor.

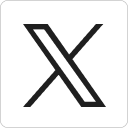



