The last few years have seen a number of interesting developments involving satellites. For instance, Planet Labs have developed a small, agile imaging satellite that allows swarms of satellites to orbit Earth, thus providing a better breadth and depth of coverage than more traditional imaging satellites.
Or you’ve got Orbital Insight, who are using satellites combined with AI to help us tackle things like deforestation. It works by keeping an eye out for suspicious changes, such as new road building around forests for instance. Once such changes have been spotted, an alert is published on the Global Forest Watch website, who are partnering with Orbital Insight.
Mapping poverty
One of the more interesting however comes from a Stanford led team, who are using a combination of satellite imaging and machine learning to better identify poverty zones in the developing world.
The team, who documented their approach in a recently published paper, aim to develop detailed poverty maps to support aid organizations with their work in the developing world.
They use machine learning to extrapolate vital information from high-res satellite imagery to help fill in some of the gaps of previous methods, which often involve labor intensive surveys of villages.
“We have a limited number of surveys conducted in scattered villages across the African continent, but otherwise we have very little local-level information on poverty,” the researchers say “At the same time, we collect all sorts of other data in these areas – like satellite imagery – constantly.”
Spotting patterns
Satellite images are in ready supply, so the team set out to test whether they could extrapolate useful information from them. With little poverty data to feed the algorithm, machine learning at first appeared a dead end.
“There are few places in the world where we can tell the computer with certainty whether the people living there are rich or poor,” the team explain. “This makes it hard to extract useful information from the huge amount of daytime satellite imagery that’s available.”
So they set about using more left-field data instead, such as the amount of light displayed at night, to make educated guesses about the income levels of the area.
When the algorithm was fed this information, it was easily able to pick out both the lighting levels of an area, but also important features such as roads and farmland, and was subsequently adept at predicting wealth levels of a village.
Indeed, the algorithm was so effective that it outperformed the more traditional methods used to create similar poverty maps.
“Our paper demonstrates the power of machine learning in this context,” the team conclude. “And since it’s cheap and scalable – requiring only satellite images – it could be used to map poverty around the world in a very low-cost way.”
Check out the video below to see the algorithm in action.

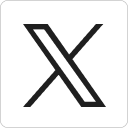



