I’ve written before about the apparent shift in healthcare whereby making sense of the vast quantities of data produced within the system is key to successful treatment of patients.
Nowhere is this more so than in cancer care. For instance, a team from UCL utilized deep learning earlier this year to more accurately identify cancer cells.
This trend is continued with a second study, which aims to make sense of the cancer data currently sitting in the cancer registry program that’s coordinated by the National Cancer Institute (NCI) and the Centers for Disease Control and Prevention. This database has records of cancer incidences across the US, but the curation of it can often be a hugely time-intensive process as it requires manual editing and annotation from experts for each file.
Automated assistance
“The manual model is not scalable,” the team say. “We need to develop new tools that can automate the information-extraction process and truly modernize cancer surveillance in the United States.”
The researchers have been working on this for a few years, but have made considerable progress since adopting deep learning. They developed algorithms to search for and extract valuable information from the reports in the database. When tested on even a small sample, the system proved very capable of uncovering some previously hidden insights.
“Today we’re making decisions about the effectiveness of treatment based on a very small percentage of cancer patients, who may not be representative of the whole patient population,” they say. “Our work shows deep learning’s potential for creating resources that can capture the effectiveness of cancer treatments and diagnostic procedures and give the cancer community a greater understanding of how they perform in real life.”
Creating context
The team first set about ensuring the system had a contextual understanding by feeding the neural network a load of data. The researchers accelerated this by conducted multiple calculations at the same time.
When this algorithm was programmed to perform multitask learning, its performance went up considerably, especially compared to more traditional methods.
“Intuitively this makes sense because carrying out the more difficult objective is where learning the context of related tasks becomes beneficial,” the team say. “Humans can do this type of learning because we understand the contextual relationships between words. This is what we’re trying to implement with deep learning.”
To further test the approach, the team conducted a second study on a more complex data challenge. They set out to use deep learning to connect up the origins of a cancer with a topological code, thus providing a very high level of granularity.
By using the convolutional neural networking approach typically deployed in image recognition, they were able to feed the algorithm data from a range of sources. The algorithm then created a model from this data to make connections between words.
The results from initial tests are certainly promising, and the team hope to scale up their work with larger datasets, with the algorithms performing under reduced supervision.
Becoming more adept at deriving insights from vast datasets like this is likely to be increasingly important for the quality of healthcare in future. Hopefully the lessons from these various projects are readily shared around the industry so we become collectively wiser as a result. The researchers themselves admit that they have barely scratched the surface of what can be achieved, so it will be exciting to follow progress in the coming years.

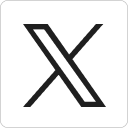



