Recently I wrote about a study that examined the use of big data to reduce hospital waiting times. The authors propose a model that translates predictions on the arrival of patients into the emergency room into a better process for dealing with those numbers.
At the moment, hospitals often use congestion data, with ambulances instructed to divert to another facility once an existing facility is too over-loaded. The authors suggest that this can be improved significantly by using predictive data so that hospital staff can predict when that congestion might occur rather than reacting to it when it does. By doing this, hospitals can divert patients before load becomes excessive, and thus reduce waiting times for patients.
“These delays can have significant, life-changing ramifications,” the authors say. “These are the kinds of changes that potentially could affect all of us.”
Smoothing admissions
The Assistance Publique-Hôpitaux de Paris (AP-HP) hospital group are attempting to put this kind of thing into practice. The group have analyzed ten years worth of data on hospital admissions, flu rates, weather and so on by its Trusted Analytics Platform (TAP). The aim is to better predict hospital admissions, and therefore better route people to the best facility.
TAP is an open source platform that uses machine learning to help analysis of data. The AP-HP project is the first to use the platform for time series analysis, but should the project be successful, it will be rolled out across all of the 44 hospitals in the group.
The system aims to provide admissions staff with accurate predictions up to 15 days in advance, thus hopefully providing sufficient warning to allow suitable staffing and other resources to be on hand in periods of high demand.

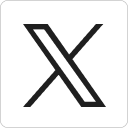



