I’ve written before about how data is increasingly being used by transport planners to manage the networks within their cities. A good example comes from Montreal, where they’ve teamed up with traffic management company Orange Traffic to install around 100 sensors on the busiest roads in the city. The sensors are designed to detect Bluetooth signals from the mobile phones of drivers, and thus provide planners with a detailed, yet inexpensive method of monitoring traffic flow in the city.
By tracking the vehicle through multiple sensors, the team are able to detect the speed of the traffic as well as its direction. The data is sent to the Urban Mobility Management Center where it is analyzed to help ensure good traffic flow.
Efficient transit
Researchers from A*STAR have developed an algorithm to accurately recreate and predict public transport usage in Singapore. The model, which is based upon land-use patterns in the city, aims to ease the flow of commuters in and out of the city each day.
The government of Singapore are aiming to alleviate the pressures during the commute by shifting away from the traditional concentric ring approach to urban planning towards more regional centers of commerce.
“We’re aiming to understand the recipe for a smart city,” the researchers explain. “Singapore needs an efficient transport system to support people’s activities given the existing and planned infrastructure. To guide planners, we needed a model that could predict ridership under the regional centers plan.”
The data behind the project originated from the smartcard system use whenever they access the public transport network. Over the week long research period, this amounted to more than 20 million journeys.
This data was then combined with city-level land use data and high-res maps for individual amenities within a radius of the metro stations.
Finding the optimum
In total, the team used three different machine learning based models to find the most efficient network for the city, both in terms of reproducing traffic flows and predicting future ridership across the city.
“We found that a decision tree model performed best, with good accuracy, computational efficiency and an easy-to-follow user display,” the team say. “Results indicated that an increase in amenities of up to 55 per cent across the city would increase ridership. Beyond this point, ridership begins to decline; this is logical because if amenities are available locally, people walk instead.”
The team believe that the model will be crucial in guiding urban planning in Singapore in the future, and that it can be equally adept when applied to other cities as well. It’s another example of how data can be influencing urban transportation.

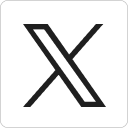



