The last year or so has seen a number of fascinating projects using AI to help our understanding of the physical world. Mostly these projects have revolved around monitoring vibrations to infer the health of a material.
You also have, however, the Material Genome Project, which aims to predict the lifespan of any machine.
Of a similar bent is a recent project from researchers at the Lawrence Berkeley National Laboratory, whereby algorithms have been developed that can predict defects in certain intermetallic compounds with extremely high levels of accuracy.
Advanced alloys
The hope is that it will support the development of advanced alloys and lightweight materials for industries ranging from aerospace to automotive.
The researchers remind us that all materials have some form of defect, which underpins the properties they exhibit. These defects may appear as vacancies, which are essentially ‘holes’ in the substance’s crystal structure, or antisite defects, which are essentially atoms placed on the wrong crystal site.
The more we can understand such defects, the better we are able to design materials that have structural stability and strength. This has traditionally been done by using density functional calculations, but the approach is very expensive to perform, and therefore is limited in its application.
“Density functional calculations work well if you are modeling one small unit, but if you want to make your modeling cell bigger the computational power required to do this increases substantially,” the researchers say. “And because it is computationally expensive to model defects in a single material, doing this kind of brute force modeling for tens of thousands of materials is not feasible.”
Computational challenges
To overcome these computational obstacles, the teams used machine learning to more efficiently predict defects in intermetallic compounds. They tested the algorithm out on 100 distinct compounds, running density function calculations to test for defects.
“This work is essentially a proof of concept. It shows that we can run density functional calculations for a few hundred materials, then train machine learning algorithms to accurately predict point defects for a much larger group of materials,” the team say.
“The benefit of this work is now we have a computationally inexpensive machine learning approach that can quickly and accurately predict point defects in new intermetallic materials “ they continue. “We no longer have to run very costly first principle calculations to identify defect properties for every new metallic compound.”
They hope that it will eventually offer a fast and effective way to predict defects, which will in turn accelerate the design of new materials. It will form part of the Materials Project, which is an open source initiative that provides open, web-based access to information about materials, together with an open-source, Python based toolkit to allow people to model point defects in things such as semiconductors.

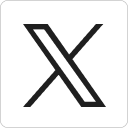



