New drugs typically take 12-14 years to make it to market, with a 2014 report finding that the average cost of getting a new drug to market had ballooned to a whopping $2.6 billion.
It’s a topic I’ve covered before, with a study published earlier this year highlighting how automation could be used to reduce the cost of drug discovery by approximately 70%.
Another example of what’s possible is provided by a recent study published in Cell Chemical Biology. The study reveals a big data based approach to detecting toxic side effects that would prohibit a drug from being used on humans before it gets to the expensive clinical trial stage.
The latest project of this ilk comes from a team from the University of Toronto, who in a recent paper describe the use of machine learning to generate 3D structures of protein molecules to assist with drug development.
“Designing successful drugs is like solving a puzzle,” the team say. “Without knowing the three-dimensional shape of a protein, it would be like trying to solve that puzzle with a blindfold on.”
Drug development
The team believe that being able to determine the atomic structure of protein molecules will play a huge role in understanding how they work, and how they may respond to drug therapies. The drugs typically work by binding to a protein molecule, and then changing its shape and thus altering how it works.
Therefore, the ideal drug binds to a specific form of protein to fight a disease, whilst not binding to those proteins not involved and causing side effects.
The algorithm reconstructs the proteins using microscopic images. The size of proteins renders them invisible unless viewed using techniques such as electron cryomicroscopy (Cryo-EM), but the new method allows for many proteins to be studied that would not have been viable in the past.
Putting the pieces together
Cryo-EM is a novel approach because it uses extremely high-powered microscope to take thousands of low-res images, with the challenge then being to piece them back together so that they make sense, which is where machine learning comes in.
“Our approach solves some of the major problems in terms of speed and number of structures you can determine,” the team say.
By speeding up the process of deriving the correct protein structure, the researchers hope that they will expedite the drug development process significantly.
“Existing techniques take several days or even weeks to generate a 3D structure on a cluster of computers,” they say. “Our approach can make it possible in minutes on a single computer.”
What’s more, the current methods can often generate incorrect structures, especially if no prior knowledge of the protein is fed into the process. The new method requires no such prior knowledge to be successful.
“We hope this will allow discoveries to happen at a ground-breaking pace in structural biology,” they conclude. “The ultimate goal is that it will directly lead to new drug candidates for diseases, and a much deeper understanding of how life works at the atomic level.”

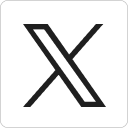



