I’ve written a few times previously about the growing volume of data in healthcare, and the tremendous possibilities this presents as we grow our capabilities to make sense of it.
A recent McKinsey report highlights the huge potential for data to improve operations, but securing the skills and capabilities to capitalize on the data held is a significant problem. Indeed, a recent report from CrowdFlower found that 83% of companies lack the data science skills they need.
This is certainly the case in the National Health Service, where a recent report from GovLab reveals a significant lack of personnel with data analytical skills.
The paper makes a number of recommendations to improve capacity within the NHS, with these suggestions falling into four main categories:
- The use of technology to better coordinate the talent already present in the NHS, with the utilization of things such as marketplaces.
- Using skills banks to allow project teams to better understand the talent they have at their disposal.
- Use expert networks to better connect with talent sitting outside of the NHS.
- Using mechanisms such as challenge prizes to allow the NHS to bring in talent from external sources more effectively.
Data support
Whilst these are undoubtedly worthy suggestions and ones I hope the NHS will take up, there are a number of other possible avenues they can explore.
For instance, there’s one thing making the skillsbase deeper, but software can also be used to make data analysis easier. For instance, Beautiful Information is a startup that achieved support from UK innovation charity Nesta in 2016. They have developed an Operational Control Centre app that aims to present data in a more accessible way.
The app aims to transform previously unmanageable data into usable information by displaying it in a visual and real-time way to both managers and clinicians. The app comes with a customizable dashboard so teams and organizations can gain access to the exact data they desire, whether that’s patient waiting time or the throughput of a particular department.
The hope is that it makes data based decision making accessible to people who aren’t trained data scientists.
Using automation
There are also tools being developed to help automate much of the triaging process so that data scientists can focus on what really matters.
For instance, a team of MIT researchers have developed an automated tool to do just that. The researchers recently published a couple of papers on the process, including the preparation of data and even the creation of problem specifications.
“The goal of all this is to present the interesting stuff to the data scientists so that they can more quickly address all these new data sets that are coming in,” the authors say. “[Data scientists want to know], ‘Why don’t you show me the top 10 things that I can do the best, and then I’ll dig down into those?’ So [these methods are] shrinking the time between getting a data set and actually producing value out of it.”
The researchers, who are bringing their tool to market via their Feature Labs company, developed a new programming language, called Trane, to reduce the time data scientists spend on defining prediction problems to days rather than months. The team are confident that similar improvements can be made for label-segment featurize (LSF) processes.
The system was tested out on real-world questions that were posed by data scientists working with around 60 datasets. Even with a relatively small sample, the system was capable of devising not only all of the questions posed by the data scientists, but also many that they hadn’t considered.
These are all ways in which the data science capacity in the NHS could be expanded, and with data from mobile, wearables, IoT and genomics set to grow exponentially in the coming years, these appear rather more scaleable than increasing the number of human data scientists available.
Before I leave, the European Commission recently published a paper on the policy implications of big data in healthcare, which I covered in a previous post. If health data is a topic of interest, you might wish to check it out.

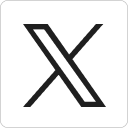



