Predicting extreme events is far from straight forward, and I wrote recently about an AI driven attempt to predict earthquakes with greater accuracy. These technological aides are crucial as expertise is in something of a crisis at the moment, with the populist movements around the world decrying the validity of experts in our midst.
Much of this criticism revolves around the apparent inability of experts to predict significant events such as the financial crisis of 2007, with the minority of economists that did foresee it lauded for their predictive capabilities.
Whilst this is a simple and easy to understand narrative, it has a number of unexpected and undesirable side-effects that will eventually discredit even the best forecasts.
Seeing the future
A recent paper set out to explore how this kind of scenario could be avoided. The authors use theoretical arguments alongside a series of simulations based upon real economic data.
The authors argue that evaluation of forecasts typically only occurs in public when a major event has taken place, with this discussion typically occurring when the forecasters failed to predict it. A famous example of this was after the earthquake in L’Aquila that killed 309 people, after which six Italian seismologists were convicted of involuntary manslaughter.
The paper contends that the media are ill-placed to evaluate the accuracy of such forecasts, not least because by limiting the range of forecasts evaluated to the most extreme of events limits our ability to evaluate ones forecasting capabilities because it makes it rational to predict disaster on a regular basis.
“In a nutshell, if forecast evaluation is conditional on observing a catastrophic event, predicting a disaster every time becomes a worthwhile strategy,” the authors say.
Here comes Cassandra
This forecaster’s dilemma infers that because the media focuses almost solely on extreme events, it makes it tempting to base ones decision making on such misguided inferential procedures.
So how might one overcome this? The authors argue that method is key. If forecasts take the form of probability distributions, then the authors believe such conflicts may be overcome, although of course, the media only tend to trot out forecasters that are overwhelmingly certain about their predictions.
It brings to mind the famous superforecasting work led by Philip Tetlock a few years ago. The researchers recruited a team of over a thousand forecasters from places such as professional bodies, research institutions and even blogs (although not this one I should add).
Every two weeks this pool of people were asked questions that elicited their thoughts on various events, which they input on the projects website.
The team ran a series of experiments to try and improve the forecasts from the collective. Three core factors emerged from these experiments.
- Training on how to make good predictions helped
- Working collectively rather than independently was also found to support good predictions
- Tracking performance was also useful, as it allowed ‘super teams’ to be born of the best forecasters, with the best performers driving each other on even more
Forecasting is undoubtedly crucial for organizations of all kinds, and so it’s crucial that we get better at it. Whether it’s deploying AI or fine tuning our own instincts and methods, it’s good to see so many projects underway to try and make this so.

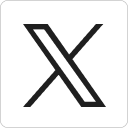



