As robots have taken on ever greater roles in warehouses and other industrial settings, their ability to grasp and manipulate fragile items has been of increasing importance. To date they have remained a considerable distance behind humans, and it has to a large extent held back the deployment of machines in such environments.
An example of the progress being made however comes via a recent study conducted by MIT’s Computer Science and Artificial Intelligence Laboratory (CSAIL), in which robots are capable of inspecting random objects, and then visually understanding them to a high enough level to accomplish certain specific tasks.
Dense Objects Net
The system, which is called Dense Objects Net (DON), aims to examine objects via a collection of points that help it create a virtual roadmap. The team believe this approach allows the robot to understand and manipulate objects, even when they are in a pile of similar looking items.
“Many approaches to manipulation can’t identify specific parts of an object across the many orientations that object may encounter,” the researchers explain. “For example, existing algorithms would be unable to grasp a mug by its handle, especially if the mug could be in multiple orientations, like upright, or on its side.”
Whilst many of the applications for this technology are in industrial settings, the team also believe it could have applications in the home too. The fact that the robot was able to learn in a self-supervised way makes it particularly interesting, as humans weren’t required to annotate the data.
Training the machine
The system was trained to examine objects as a series of points so that it can construct a larger coordinate system. This can then be used to plot different points together and form a 3D visualization of each object.
The team believe that their work is superior to existing systems, such as UC-Berkeley’s DexNet, because it’s capable of satisfying specific requests. The researchers liken it to an 18-month old child who can grab lots of toys versus a four-year old child who can grab specific toys by a specific part.
The system was put through its paces on a soft caterpillar toy, with ON able to grasp the right ear of the toy from a number of different configurations. It was also tested on various baseball hats, and again, DON was able to specify the target despite the hats having very similar designs, and despite having never seen the hats in any kind of training data set.
“In factories robots often need complex part feeders to work reliably,” the researchers explain. “But a system like this that can understand objects’ orientations could just take a picture and be able to grasp and adjust the object accordingly.”
They next hope to continue improving the system so that it can perform specific tasks with an even deeper level of understanding of the objects its working with. It’s a fascinating project, and you can see DON in action via the video below.

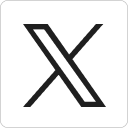



