When I was studying AI as part of my MSc in computing 15 years or so ago, AI still largely had a terrible reputation. It had suffered from decades of over-promise and under-delivery, and if you went into a company saying you wanted to do something with AI, you’d be treated with a high degree of scepticism.
Now, it’s rare to find any technology-driven venture that doesn’t have AI of some kind in it. It’s akin to the dot-com boom whereby every single business in the world, and indeed most projects within businesses, had to have a web element to it. Now, the same is largely the case with AI.
At the recent O’Reilly AI Conference, Northwestern University’s Kris Hammond suggested there are three drivers for this. The first is that AI has been puffed up to be godlike in its capabilities. Headlines project a technology that will destroy jobs, disrupt industries and generally have a profound and worrying impact upon us as individuals, as organizations and even as society as a whole.
This creates a sense of fear that is largely joined with a fear of being left behind. Just as headlines push the capabilities of AI to do great things, they also frequently remind us that most of us are relative laggards. Statistically, this can’t, of course, be true, and while the Facebook’s, Amazon’s and Google’s of the world are indeed doing great things, most companies are not at all.
This fear is compounded by a generally high-level of ignorance around what AI is, what it can do, and how it can benefit the business. Without this understanding it’s impossible to take an intelligent perspective on what AI can do for your business, so instead executives just strive to do something, anything to ensure they’re not ‘that’ organization that is not using AI to ‘transform’ their business.
How to integrate AI into the enterprise
So how can organizations overcome this? Overcoming ignorance is a good place to start, and the tutorial given by Hammond was a pleasing break from many technology events that are largely attended by people from whatever discipline the event covers. Data scientists attend data events, roboticists attend robotics events, and so on. At the O’Reilly event however, techies were in the minority, with most of the attendees from managerial functions.
The session began by providing an overview of what AI is, with a whistle stop tour of machine learning, and specifically the nature of learning itself, which feeds into the supervised, unsupervised and reinforcement learning models used by all machine learning systems today.
Machine learning is, of course, just one aspect of AI, with McKinsey recently identifying five distinct forms, including physical AI, computer vision, natural-language processing, natural language, and then machine learning. Understanding what each of these is, even on a basic level, can you help you to make informed choices, and not be suckered in by hype.
From this basis, you can look at the core challenges you have in your business and explore whether AI can help you. Hammond recommends three things to consider when assessing whether a problem is suitable for AI:
- Is your task genuinely data driven?
- Do you have suitable and sufficient data to support any AI-driven solution?
- Do you need the scale that automation provides?
If you’re presented with solutions that ignore the business, ignore the data, are technology orientated and that put promises before the problem, then that should set warning signals firing.
Putting the cart before the horse
In many ways we can greatly benefit from stripping the hype from AI, and indeed from the other hot topics of our age (blockchain perhaps, or quantum computing). This hype causes us to have excessive expectations around just what the technology can, or should, achieve.
As far as AI is concerned, perhaps a more sensible, if less sexy, first step is to begin building the data foundations upon which AI undoubtedly rests. If you don’t have a robust data analytics function, then it will be very hard to build any more comprehensive AI functions at a later date.
From that, the next challenge is one of mindset. Sure, having the technologies and skills to do this is crucial, but there is an important part that is not technology related at all. It’s tempting when you go to events like the O’Reilly one to think that managers need to know the data, the maths and the AI themselves in order to be effective.
In reality however, you will have data scientists and coders to do the technical stuff, just as you’ll have people on the business side who are experts in what they do. What managers need to be is able to translate between the two worlds.
So sure, some level of understanding of the technology is required. It’s not enough to simply ‘trust’ the technology like it’s a black box, but neither do you need to be an expert yourself. What you need is enough of an understanding to help you start collecting and managing the size of datasets that will be required to drive your business.
I suspect, much as the web did before, that AI will eventually transform every business, but that it will take a bit longer than we expect. As a leader, your role is to ensure that the technologists aren’t in sole control of your AI work, and that they remain tethered to the business. If you can provide that conduit and ensure that the organization works on its most pressing problems, then you have a much better chance of success.
It’s perhaps no surprise however that in surveys of adoption, the most advanced companies in terms of AI are also the most advanced in other areas of digitization. The early adopters of AI are the ones who have invested in the digital capabilities, and the evidence to date suggests that it’s simply not possible to leapfrog to AI without first doing the ‘basic’ digital transformation work. If you haven’t started yet, there’s no better time than today.

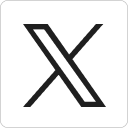



