Whenever you launch a new product it can often be difficult to accurately gauge demand such that you can produce just the right amount of product. This is especially difficult when you’re producing something completely new and therefore don’t even have rivals to judge demand from.
A new study from Northwestern aims to explore how data and AI can do a better job of accurately predicting product demand. This can be especially difficult with technology products that can often spike in the early months before flattening off significantly.
To overcome the fundamental lack of data associated with truly novel products, the team harvested data about existing products with similar features, with these acting as a proxy for older generations of the new product. The tool aims to go beyond just providing data however.
“But beyond the data component, we also allow the top manager to overlay their perspective or their feelings for this new product,” the researchers explain. “For example, if they think this is going to be a great product, they would probably do more promotional events, which will change some of the demand patterns along the way. So we also allow our forecast to be flexible enough to incorporate those business insights such as the planned launch time, promotional campaigns or planned sales events.”
A blended approach
The team believe that this blended approach can effective forecast demand even for products without much in the way of data behind them. The team tested their model on data from various Dell products, who partnered with the team on the research. The model was able to improve official Dell forecasts by around 3.4% for brand-new products, 9.2% for relatively new products and 14% for established products. The savings from this are substantial, with up to $4.70.
What’s more, these savings can be even bigger at smaller firms who lack the kind of resources a company like Dell can utilize on forecasting. For instance, when the team tested their system at startup Turtle Beach, the forecast accuracy increased by an enormous 73%.
The model was primarily developed for tech products, but the team believe it can be equally valuable for all kinds of product, and even those with varying lifecycles, such as fast fashion, which require a tremendously quick payback.
“The methodology is universal,” the team conclude. “But the need for this framework becomes more urgent when the product life cycle is short, so companies can really prepare for that one shot of sales.”

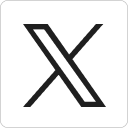



