Radiation therapy has undoubtedly been revolutionary in its treatment of cancer. The process is far from fail-safe however, and requires the oncologist to accurately mark off the tumor for the treatment to be applied. It’s a process that requires high levels of skill, and can be time consuming in even the most developed of countries. In less developed countries where personnel are lacking in expertise and experience, the process can be nay on impossible.
Recent research from Harvard explored whether AI could do the job as effectively as a trained oncologist. Central to the project was a crowdsourcing competition that saw 34 contestants competing to analyze around 78,000 radiology images alongside 45 different AI algorithms. The project found that, as is so common, the best results were achieved when man and machine worked in unison.
“A combined crowd innovation and AI approach rapidly produced automated algorithms that replicated the skills of a highly trained physician for a critical task in radiation therapy,” the authors explain.
Crowd innovation
The researchers believe that image-related work in healthcare is ideally suited to crowd-based innovation, and success can be achieved even when participants are non-domain experts. That was illustrated by this project, which was hosted on the coding community Topcoder. Whilst they lack medical knowledge, many members are experts in image analysis in other fields.
It’s an approach the researchers believe can easily apply to other biomedical problems, but the shortage of well-annotated images is a major impediment at the moment, both to crowdsourcing and to AI-based projects. We are also held back by regulatory hurdles related to privacy and de-identification of data.
Perhaps the biggest challenge however is identifying what the gold standard actually looks like to measure entrants against. Many medical settings prevent such neat conclusions from being drawn, with diagnoses instead being very subjective to the particular circumstances.
Whilst crowdsourcing does promise to speed up the innovation process, this is only up to a point, as securing FDA approval for any diagnostic test still takes a considerable length of time. It’s not immediately clear whether the researchers are prepared to stick around to make that happen or whether their motivations are more to prove the merits of open innovation and crowdsourcing in a new theater. My suspicion is it’s more the latter, and they will be moving onto new applications of the approach rather than taking this one to market, which is a little bit frustrating as the true value of any innovation only arrives when it’s helping real people in real situations. Nonetheless, it does reinforce the potential of crowdsourcing to make an impact in healthcare, even if the hard work is still to come.

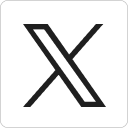



