The application of machine learning in healthcare has been widespread in recent years, but one of the more interesting use cases has been in the field of mental health. A range of projects have attempted to diagnose conditions such as depression faster and more accurately than is currently the case. The latest project in this ilk comes from new research from Indiana University, which showcases how machine learning is capable of predicting which patients may need more treatment for depression.
The researchers highlight just how prevalent depression is, with some 350 million people estimated to suffer from it by the World Health Organization. Some are able to self-manage their condition, but others require more advanced support from mental health care providers. It was these more challenging cases that the researchers focused on, with their algorithm aiming to better identify them so they can get the help they need.
“Our goal was to build reproducible models that fit into clinical workflows,” the researchers explain. “This algorithm is unique because it provides actionable information to clinicians, helping them to identify which patients may be more at risk for adverse events from depression.”
The algorithms were trained on a range of behavioral and clinical data from the Indiana Network for Patient Care. The dataset contained information from a diverse patient population to allow for robust models to be created that are feasible for use in a range of clinical environments.
“By creating models for different patient populations, we offer health system leaders the option of selecting the best screening approach for their needs,” the authors say. “Perhaps they don’t have the computational or human resources to run models on every single patient. This gives them the option to screen select high-risk patients.”
Practical use
The authors remind us that primary care doctors are often incredibly time poor, so require the ability to identify patients with the severest form of depression both reliably and rapidly. They believe that their approach provides both of these things, not least because it’s able to tap into the increasingly rich data available to bioinformaticians.
They hope to continue to develop their model, and integrate a range of additional social determinants of health into them to make them even more robust. The ultimate aim is to get the model into the hands of clinicians so that they can start supporting patients with the strongest signs of depression more effectively.

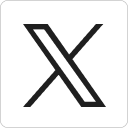



