AI has proven remarkably adept at predicting various health issues ahead of time, and a new study from MIT’s Computer Science and Artificial Intelligence Laboratory (CSAIL) shows how adroit it is at understanding the risk we face of cardiovascular death.
The system, which they’ve dubbed ‘RiskCardio’, is targeted to people who have survived an accute coronary syndrome (ACS), which covers a wide range of conditions that involve a reduction or blockage in the blood flow to the heart. It relies upon around 15 minutes worth of data from an electrocardiogram (ECG) to produce a risk score for the individual.
Those in the upper quartile of the risk register were around seven times more likely to die of cardiovascular issues than those in the lowest quartile. This may sound obvious, but in current risk metrics, that differential is only around 3x as great, which suggests the RiskCardio app is slightly better at detecting the most at risk groups.
“We’re looking at the data problem of how we can incorporate very long time series into risk scores, and the clinical problem of how we can help doctors identify patients at high risk after an acute coronary event,” the researchers say. “The intersection of machine learning and healthcare is replete with combinations like this — a compelling computer science problem with potential real-world impact.”
Judging risk
RiskCardio differs from previous models in that it only uses ECG data rather than things such as age and weight. The system distinguishes the patient’s ECG data and looks for variability between individual beats to predict risk in the future.
The system is so proficient that it is capable of accurately gauging risk after just 15 minutes worth of data, with the prediction accurate to within 30 days.
The team hope to continue improving the system by training it on a more diverse and inclusive dataset to take account of different ages, genders and ethnicities. They also want to take account of practical issues around poorly labeled or unlabeled data, and explore how the system can cope with that information and still function well.
“Machine learning is particularly good at identifying patterns, which is deeply relevant to assessing patient risk,” they conclude. “Risk scores are useful for communicating patient state, which is valuable in making efficient care decisions.”

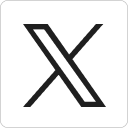



