Failure has seldom been sexier in terms of the acceptance of it in working life. Advocates argue that not only is failure an expression of truly pushing our boundaries, but also provides us with a great opportunity to learn and develop, both as individuals and as teams.
Failing in and of itself is not always the answer of course, as new research from the University of Arizona reminds us. The authors remind us that there is usually a sweetspot, in which a task is just hard enough to stretch us, but not so challenging that we fail all the time and are therefore more likely to give up.
They believe this sweetspot occurs when we fail around 15% of the time in our endeavors, with a success rate therefore of around 85%. This is when the best learning appears to emerge.
“These ideas that were out there in the education field – that there is this ‘zone of proximal difficulty,’ in which you ought to be maximizing your learning – we’ve put that on a mathematical footing,” the researchers say.
The 85% rule
The 85% figure emerged after the team conducted a number of machine-learning experiments whereby computers were taught a number of straightforward tasks, including the classification of different patterns or photographs. The computers appeared to learn best when the success rate was around the 85% mark.
“If you have an error rate of 15% or accuracy of 85%, you are always maximizing your rate of learning in these two-choice tasks,” the authors say.
This was then compared with previous studies into learning among animals, and a similar figure emerged in those instances too. It’s a ballpark that the researchers believe is most applicable in perceptual learning, in which we learn gradually from our experiences and from examples. They cite the example of a radiologist learning the difference between scans with tumors present and those without.
“You get better at figuring out there’s a tumor in an image over time, and you need experience and you need examples to get better,” they explain. “I can imagine giving easy examples and giving difficult examples and giving intermediate examples. If I give really easy examples, you get 100% right all the time and there’s nothing left to learn. If I give really hard examples, you’ll be 50% correct and still not learning anything new, whereas if I give you something in between, you can be at this sweet spot where you are getting the most information from each particular example.”
The researchers accept that their work has various limitations, not least the relative simplicity of the tasks they were assessing. It’s by no means certain that the same heuristic applies for more complex tasks, but it is an area that they believe warrants further exploration to see whether the heuristic holds up across a variety of tasks.

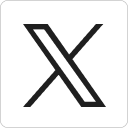



