One of the most challenging conversations a healthcare professional can have is when discussing life threatening illnesses and procedures. It can be a delicate balance between fully explaining what’s to come whilst remaining sensitive to the intensity of what people are being told.
It’s perhaps not the most natural theater for machine learning to play a part, but new research from the University of Vermont suggests machine learning and natural language process can help us to better understand these conversations, which in turn can help healthcare providers to improve their end-of-life communications.
The researchers borrowed an approach more commonly seen when assessing works of fiction, with a machine learning algorithm analyzing the language used and determining the different types of stories. The approach was adapted to enable it to successfully assess over 350 transcripts of palliative care conversations.
Each conversation was broken into 10 parts, each with the same number of words in. The texts were assessed to determine the frequency and distribution of words, especially those in relation to time, sentiment, illness terminology and changing moods.
Strong signals
The analysis began to detect a number of strong signals. End-of-life conversations would often progress from talking about things in the past, to talking about the future. Equally, the sentiment of the conversations would go from sad to happy, with a significant range between the two.
The conversations would also typically move from one about symptoms at the start, to treatment options in the middle part, and then the prognosis toward the end. It’s a clear indication of the narrative present in such conversations.
While the team accept that practical applications of their work remain a way off, they believe the approach has the potential for better understanding a wide range of different healthcare-related conversations. The hope is that this understanding could then help to determine what a good conversation is in each context.
“One type of conversation may lead to an ongoing need for information, while another may have an ongoing need for functional support,” the researchers say. “So one of the ways those types can help us is to identify what are the resources we are going to need for individual patients and families so that we’re not just applying the same stuff to everybody.”

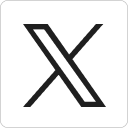



