Mental health has been one of the most blatant concerns during the Covid-19 pandemic, with stress, loneliness, and depression all rising over the past year. Diagnosing mental health issues can be difficult as people are not always keen to open up to any problems they may be facing. Researchers from the University of Birmingham believe that machine learning could play a crucial role.
Often the situation is compounded by the fact that patients with depression or psychosis rarely experience symptoms of purely one or the other illness. This has meant that mental health clinicians have had to diagnose a primary illness that also has secondary symptoms.
As such, making accurate diagnoses is often extremely challenging, and the diagnoses that are made often fail to capture the true complexity of the individual experience.
AI help
The researchers set out to explore whether machine learning might be able to help in the creation of accurate models of “pure” forms of both illnesses, and therefore aid in the accuracy of diagnoses of mental health patients, and especially those with mixed symptoms.
“The majority of patients have co-morbidities, so people with psychosis also have depressive symptoms and vice versa,” the researchers say. “That presents a big challenge for clinicians in terms of diagnosing and then delivering treatments that are designed for patients without co-morbidity. It’s not that patients are misdiagnosed, but the current diagnostic categories we have do not accurately reflect the clinical and neurobiological reality.”
The researchers recruited 300 volunteers from the PRONIA study, a European Union-funded cohort study taking place across seven European research centers. The volunteers completed questionnaires as well as clinical interviews, while data was taken from MRI scans of each person.
Small subgroups of patients within this cohort were identified as being classified as suffering from either depression without any psychotic symptoms, or psychosis without any depression symptoms.
Pure illnesses
This data allowed the team to develop machine learning models of both pure depression and pure psychosis that were used with patients with symptoms of both illnesses. The ambition was to develop an extremely accurate disease profile for each patient and then test this against their diagnosis to understand how accurate it was.
The project found that people with depression as the primary illness were more likely to receive an accurate diagnosis, whereas those with psychosis with depression had symptoms more frequently tended towards the depression dimension. This may indicate that depression plays a greater part in the illness than had previously been thought.
“There is a pressing need for better treatments for psychosis and depression, conditions which constitute a major mental health challenge worldwide,” the researchers explain. “Our study highlights the need for clinicians to understand better the complex neurobiology of these conditions, and the role of ‘co-morbid’ symptoms; in particular considering carefully the role that depression is playing in the illness.”
“In this study, we have shown how using sophisticated machine learning algorithms which take into account clinical, neurocognitive, and neurobiological factors can aid our understanding of the complexity of mental illness. In the future, we think machine learning could become a critical tool for an accurate diagnosis. We have a real opportunity to develop data-driven diagnostic methods – this is an area in which mental health is keeping pace with physical health and it’s really important that we keep up that momentum.”

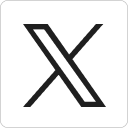



