Language barriers are a constant challenge for most migrants, but especially so for refugees and asylum seekers, who often have to explain extremely traumatic circumstances to people who don’t speak the same language. This is especially so for people who don’t speak common languages.
Researchers from the USC Viterbi School of Engineering are working on a machine learning model that can help translate for indigenous asylum seekers who speak a range of traditional languages, and thus tackle the extensive backlog that exists in the immigration system.
Communicating effectively
When asylum seekers are interviewed by the Department of Homeland Security, it’s vital that they’re able to effectively communicate the threat they face. This significantly affects their likelihood of success, with asylum seekers without lawyers prevailing just 13% of the time, compared to 74% for those with assistance.
The researchers are developing a machine translation system that works with Mexican and Central American Indigenous languages to provide legal aid to refugees and asylum seekers. The traditional approach of using parallel data is difficult as there isn’t really sufficient data for Indigenous languages, despite them being spoken by huge numbers of people. If there is no commercial reason to create parallel data, then it doesn’t tend to get created.
This is obviously not ideal for deep learning models that require huge quantities of data. Indeed, for the language the researchers are working on, they only have 15,000 sentences of parallel data for English and just 8,000 for Spanish.
Efficient training
The researchers are attempting to get around this by teaching the model to perform the task of translation before then asking it to tackle specific languages.
“It’s the same principle as learning to drive a bus by learning to drive a car first,” the researchers explain.
So they began by using a model designed to translate from English to Spanish, before modifying it to work for their Indigenous languages via an approach called transfer learning. This was then combined with old grammar books to teach the models the basics of grammar.
The project is obviously not a silver bullet for fixing the often vast problems facing the asylum system globally, but it might go a small way towards making the process easier. The researchers plan to next explore how their system could fit into the workflow of legal aid organizations.

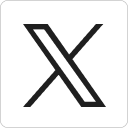



